Lower Columbia River Fish Population Indexing Analysis 2018
The suggested citation for this analytic report is:
Thorley, J.L. and Dalgarno, S. (2019) Lower Columbia River Fish Population Indexing Analysis 2018. A Poisson Consulting Analysis Appendix. URL: https://www.poissonconsulting.ca/f/1169613097.
Background
In the mid 1990s BC Hydro began operating Hugh L. Keenleyside (HLK) Dam to reduce dewatering of Mountain Whitefish and Rainbow Trout eggs.
The primary goal of the Lower Columbia River Fish Population Indexing program is to answer two key management questions:
What are the abundance, growth rate, survival rate, body condition, age distribution, and spatial distribution of subadult and adult Whitefish, Rainbow Trout, and Walleye in the Lower Columbia River?
What is the effect of inter-annual variability in the Whitefish and Rainbow Trout flow regimes on the abundance, growth rate, survival rate, body condition, and spatial distribution of subadult and adult Whitefish, Rainbow Trout, and Walleye in the Lower Columbia River?
The inter-annual variability in the Whitefish and Rainbow Trout flow regimes was quantified in terms of the percent egg dewatering as greater flow variability is associated with more egg stranding.
Methods
Data Preparation
The fish indexing data were provided by Okanagan Nation Alliance and Golder Associates in the form of an Access database. The discharge and temperature data were obtained from the Columbia Basin Hydrological Database maintained by Poisson Consulting. The Rainbow Trout egg dewatering estimates were provided by CLBMON-46 (Irvine, Baxter, and Thorley 2015) and the Mountain Whitefish egg stranding estimates by Golder Associates (2013).
Discharge
Missing hourly discharge values for Hugh-Keenleyside Dam (HLK), Brilliant Dam (BRD) and Birchbank (BIR) were estimated by first leading the BIR values by 2 hours to account for the lag. Values missing at just one of the dams were then estimated assuming \(HLK + BRD = BIR\). Negative values were set to be zero. Next, missing values spanning \(\leq\) 28 days were estimated at HLK and BRD based on linear interpolation. Finally any remaining missing values at BIR were set to be \(HLK + BRD\).
The data were prepared for analysis using R version 3.6.1 (R Core Team 2018).
Data Analysis
Model parameters were estimated using hierarchical Bayesian methods. The parameters were produced using JAGS (Plummer 2015) and STAN (Carpenter et al. 2017). For additional information on Bayesian estimation the reader is referred to McElreath (2016).
The one exception is the length-at-age estimates which were produced using the mixdist R package (Macdonald 2012) which implements Maximum Likelihood with Expectation Maximization.
Unless indicated otherwise, the Bayesian analyses used normal and uniform prior distributions that were vague in the sense that they did not constrain the posteriors (Kery and Schaub 2011, 36). The posterior distributions were estimated from 1500 Markov Chain Monte Carlo (MCMC) samples thinned from the second halves of 3 chains (Kery and Schaub 2011, 38–40). Model convergence was confirmed by ensuring that \(\hat{R} \leq 1.05\) (Kery and Schaub 2011, 40) and \(\textrm{ESS} \geq 150\) for each of the monitored parameters (Kery and Schaub 2011, 61). Where \(\hat{R}\) is the potential scale reduction factor and \(\textrm{ESS}\) is the effective sample size (Brooks et al. 2011).
The parameters are summarised in terms of the point estimate, standard deviation (sd), the z-score, lower and upper 95% confidence/credible limits (CLs) and the p-value (Kery and Schaub 2011, 37, 42). For ML models, the point estimate is the MLE, the standard deviation is the standard error, the z-score is \(\mathrm{MLE}/\mathrm{sd}\) and the 95% CLs are the \(\mathrm{MLE} \pm 1.96 \cdot \mathrm{sd}\). For Bayesian models, the estimate is the median (50th percentile) of the MCMC samples, the z-score is \(\mathrm{mean}/\mathrm{sd}\) and the 95% CLs are the 2.5th and 97.5th percentiles. A p-value of 0.05 indicates that the lower or upper 95% CL is 0.
Where relevant, model adequacy was confirmed by examination of residual plots for the full model(s).
The results are displayed graphically by plotting the modeled relationships between particular variables and the response(s) with the remaining variables held constant. In general, continuous and discrete fixed variables are held constant at their mean and first level values, respectively, while random variables are held constant at their typical values (expected values of the underlying hyperdistributions) (Kery and Schaub 2011, 77–82). When informative the influence of particular variables is expressed in terms of the effect size (i.e., percent change in the response variable) with 95% confidence/credible intervals (CIs, Bradford, Korman, and Higgins 2005).
The analyses were implemented using R version 3.6.1
(R Core Team 2018) and the
mbr
family of packages.
Model Descriptions
Condition
The expected weight of fish of a given length were estimated from the data using a mass-length model (He et al. 2008). Key assumptions of the condition model include:
- The expected weight is allowed to vary with length and date.
- The expected weight is allowed to vary randomly with year.
- The relationship between weight and length is allowed to vary with date.
- The relationship between weight and length is allowed to vary randomly with year.
- The residual variation in weight is log-normally distributed.
Only previously untagged fish were included in models to avoid potential effects of tagging on body condition. Preliminary analyses indicated that the annual variation in weight was not correlated with the annual variation in the relationship between weight and length.
Growth
Annual growth of fish were estimated from the inter-annual recaptures using the Fabens method (Fabens 1965) for estimating the von Bertalanffy growth curve (von Bertalanffy 1938). This curve is based on the premise that:
\[ \frac{\text{d}L}{\text{d}t} = k (L_{\infty} - L)\]
where \(L\) is the length of the individual, \(k\) is the growth coefficient and \(L_{\infty}\) is the maximum length.
Integrating the above equation gives:
\[ L_t = L_{\infty} (1 - e^{-k(t - t_0)})\]
where \(L_t\) is the length at time \(t\) and \(t_0\) is the time at which the individual would have had zero length.
The Fabens form allows
\[ L_r = L_c + (L_{\infty} - L_c) (1 - e^{-kT})\]
where \(L_r\) is the length at recapture, \(L_c\) is the length at capture and \(T\) is the time between capture and recapture.
Key assumptions of the growth model include:
- The mean maximum length \(L_{\infty}\) is constant.
- The growth coefficient \(k\) is allowed to vary randomly with year.
- The residual variation in growth is normally distributed.
Movement
The extent to which sites are closed, i.e., fish remain at the same site between sessions, was evaluated with a logistic ANCOVA (Kery 2010). The model estimates the probability that intra-annual recaptures were caught at the same site versus a different one. Key assumptions of the site fidelity model include:
- The expected site fidelity is allowed to vary with fish length.
- Observed site fidelity is Bernoulli distributed.
Length as a second-order polynomial was not found to be a significant predictor for site fidelity.
Length-At-Age
The expected length-at-age of Mountain Whitefish and Rainbow Trout were estimated from annual length-frequency distributions using a finite mixture distribution model (Macdonald and Pitcher 1979)
There were assumed to be three distinguishable normally-distributed age-classes for Mountain Whitefish (Age-0, Age-1, Age-2 and Age-3+) two for Rainbow Trout (Age-0, Age-1, Age-2+). Initially the model was fitted to the data from all years combined. The model was then fitted to the data for each year separately with the initial values set to be the estimates from the combined values. The only constraints were that the standard deviations of the MW age-classes were identical in the combined analysis and fixed at the initial values in the individual years.
Rainbow Trout and Mountain Whitefish were categorized as Fry (Age-0), Juvenile (Age-1) and Adult (Age-2+) based on their length-based ages. All Walleye were considered to be Adults.
Survival
The annual adult survival rate was estimated by fitting a Cormack-Jolly-Seber model (Kery and Schaub 2011, 220–31) to inter-annual recaptures of adults.
Key assumptions of the survival model include:
- Survival varies randomly with year.
- The encounter probability for adults is allowed to vary with the total bank length sampled.
Observer Length Correction
The annual bias (inaccuracy) and error (imprecision) in observer’s fish length estimates were quantified from the divergence of the length distribution of their observed fish from the length distribution of the measured fish. More specifically, the length correction that minimised the Jensen-Shannon divergence (Lin 1991) between the two distributions provided a measure of the inaccuracy while the minimum divergence (the Jensen-Shannon divergence was calculated with log to base 2 which means it lies between 0 and 1) provided a measure of the imprecision.
Capture Efficiency
The probability of capture was estimated using a recapture-based binomial model (Kery and Schaub 2011, 134–36, 384–88).
Key assumptions of the capture efficiency model include:
- The capture probability varies randomly by session within year.
- The probability of a marked fish remaining at a site is the estimated site fidelity.
- The number of recaptures is described by a binomial distribution.
Abundance
The abundance was estimated from the catch and bias-corrected observer count data using an overdispersed Poisson model (Kery and Schaub 2011, 55–56).
Key assumptions of the abundance model include:
- The capture efficiency is the point estimate from the capture efficiency model.
- The efficiency varies by visit type (catch or count).
- The lineal fish density varies randomly with site, year and site within year.
- The overdispersion varies by visit type (count or catch).
- The catches and counts are described by a gamma-Poisson distribution.
Stock-Recruitment
The relationship between the adults and the resultant number of age-1 subadults was estimated using a Beverton-Holt stock-recruitment model (Walters and Martell 2004):
\[ R = \frac{\alpha \cdot S}{1 + \beta \cdot S}\]
where \(S\) is the adults (stock), \(R\) is the subadults (recruits), \(\alpha\) is the recruits per spawner at low density (productivity) and \(\beta\) is the density-dependence.
Key assumptions of the stock-recruitment model include:
- The prior for \(\log(\alpha)\) is a truncated normal distribution from \(\log(1)\) to \(\log(5)\).
- The expected log number of recruits is affected by the egg loss.
- The residual variation in the number of recruits is log-normally distributed.
Age-Ratios
The proportion of Age-1 Mountain Whitefish \(r^1_t\) from a given spawn year \(t\) is calculated from the relative abundance of Age-1 & Age-2 fish \(N^1_t\) & \(N^2_t\) respectively, which were lead or lagged so that all values were with respect to the spawn year:
\[r^1_t = \frac{N^1_{t+2}}{N^1_{t+2} + N^2_{t+2}}\]
The relative abundances of Age-1 and Age-2 fish were taken from the proportions of each age-class in the length-at-age analysis.
As the number of Age-2 fish might be expected to be influenced by the percentage egg loss \(Q_t\) three years prior, the predictor variable \(\Pi_t\) used is:
\[\Pi_t = \textrm{log}(Q_t/Q_{t-1})\]
The ratio was logged to ensure it was symmetrical about zero (Tornqvist, Vartia, and Vartia 1985).
The relationship between \(r^1_t\) and \(\Pi_t\) was estimated using a Bayesian regression (Kery 2010) loss model.
Key assumptions of the final model include:
- The log odds of the proportion of Age-1 fish varies with the log of the ratio of the percent egg losses.
- The residual variation is normally distributed.
The relationship between percent dewatering and subsequent recruitment is expected to depend on stock abundance (Subbey et al. 2014) which might be changing over the course of the study. Consequently, preliminary analyses allowed the slope of the regression line to change by year. However, year was not a significant predictor and was therefore removed from the final model. The effect of dewatering on Mountain Whitefish abundance was expressed in terms of the predicted percent change in Age-1 Mountain Whitefish abundance by egg loss in the spawn year relative to 10% egg loss in the spawn year. The egg loss in the previous year was fixed at 10%. The percent change could not be calculated relative to 0% in the spawn or previous year as \(\Pi_t\) is undefined in either case.
Model Templates
Condition
data {
int nYear;
int nObs;
vector[nObs] Length;
vector[nObs] Weight;
vector[nObs] Dayte;
int Year[nObs];
parameters {
real bWeight;
real bWeightLength;
real bWeightDayte;
real bWeightLengthDayte;
real sWeightYear;
real sWeightLengthYear;
vector[nYear] bWeightYear;
vector[nYear] bWeightLengthYear;
real sWeight;
model {
vector[nObs] eWeight;
bWeight ~ normal(5, 5);
bWeightLength ~ normal(3, 2);
bWeightDayte ~ normal(0, 2);
bWeightLengthDayte ~ normal(0, 2);
sWeightYear ~ normal(0, 2);
sWeightLengthYear ~ normal(0, 2);
for (i in 1:nYear) {
bWeightYear[i] ~ normal(0, exp(sWeightYear));
bWeightLengthYear[i] ~ normal(0, exp(sWeightLengthYear));
}
sWeight ~ normal(0, 5);
for(i in 1:nObs) {
eWeight[i] = bWeight + bWeightDayte * Dayte[i] + bWeightYear[Year[i]] + (bWeightLength + bWeightLengthDayte * Dayte[i] + bWeightLengthYear[Year[i]]) * Length[i];
Weight[i] ~ lognormal(eWeight[i], exp(sWeight));
}
..
Block 1.
Growth
.model {
bK ~ dnorm (0, 5^-2)
sKYear ~ dnorm(0, 5^-2)
for (i in 1:nYear) {
bKYear[i] ~ dnorm(0, exp(sKYear)^-2)
log(eK[i]) <- bK + bKYear[i]
}
bLinf ~ dunif(100, 1000)
sGrowth ~ dnorm(0, 5^-2)
for (i in 1:length(Year)) {
eGrowth[i] <- (bLinf - LengthAtRelease[i]) * (1 - exp(-sum(eK[Year[i]:(Year[i] + dYears[i] - 1)])))
Growth[i] ~ dnorm(max(eGrowth[i], 0), exp(sGrowth)^-2)
}
..
Block 2.
Movement
.model {
bFidelity ~ dnorm(0, 2^-2)
bLength ~ dnorm(0, 2^-2)
for (i in 1:length(Fidelity)) {
logit(eFidelity[i]) <- bFidelity + bLength * Length[i]
Fidelity[i] ~ dbern(eFidelity[i])
}
..
Block 3.
Survival
.model{
bEfficiency ~ dnorm(0, 5^-2)
bEfficiencySampledLength ~ dnorm(0, 5^-2)
bSurvival ~ dnorm(0, 5^-2)
sSurvivalYear ~ dnorm(0, 5^-2)
for(i in 1:nYear) {
bSurvivalYear[i] ~ dnorm(0, exp(sSurvivalYear)^-2)
}
for(i in 1:(nYear-1)) {
logit(eEfficiency[i]) <- bEfficiency + bEfficiencySampledLength * SampledLength[i]
logit(eSurvival[i]) <- bSurvival + bSurvivalYear[i]
eProbability[i,i] <- eSurvival[i] * eEfficiency[i]
for(j in (i+1):(nYear-1)) {
eProbability[i,j] <- prod(eSurvival[i:j]) * prod(1-eEfficiency[i:(j-1)]) * eEfficiency[j]
}
for(j in 1:(i-1)) {
eProbability[i,j] <- 0
}
}
for(i in 1:(nYear-1)) {
eProbability[i,nYear] <- 1 - sum(eProbability[i,1:(nYear-1)])
}
for(i in 1:(nYear - 1)) {
Marray[i, 1:nYear] ~ dmulti(eProbability[i,], Released[i])
}
..
Block 4.
Capture Efficiency
.model {
bEfficiency ~ dnorm(0, 5^-2)
sEfficiencySessionAnnual ~ dnorm(0, 2^-2)
for (i in 1:nSession) {
for (j in 1:nAnnual) {
bEfficiencySessionAnnual[i, j] ~ dnorm(0, exp(sEfficiencySessionAnnual)^-2)
}
}
for (i in 1:length(Recaptures)) {
logit(eEfficiency[i]) <- bEfficiency + bEfficiencySessionAnnual[Session[i], Annual[i]]
eFidelity[i] ~ dnorm(Fidelity[i], FidelitySD[i]^-2) T(FidelityLower[i], FidelityUpper[i])
Recaptures[i] ~ dbin(eEfficiency[i] * eFidelity[i], Tagged[i])
}
..
Block 5.
Abundance
.model {
bDensity ~ dnorm(5, 5^-2)
sDensityAnnual ~ dnorm(0, 2^-2)
for (i in 1:nAnnual) {
bDensityAnnual[i] ~ dnorm(0, exp(sDensityAnnual)^-2)
}
sDensitySite ~ dnorm(0, 2^-2)
sDensitySiteAnnual ~ dnorm(0, 2^-2)
for (i in 1:nSite) {
bDensitySite[i] ~ dnorm(0, exp(sDensitySite)^-2)
for (j in 1:nAnnual) {
bDensitySiteAnnual[i, j] ~ dnorm(0, exp(sDensitySiteAnnual)^-2)
}
}
bEfficiencyVisitType[1] <- 0
for (i in 2:nVisitType) {
bEfficiencyVisitType[i] ~ dnorm(0, 2^-2)
}
sDispersion ~ dnorm(0, 2^-2)
sDispersionVisitType[1] <- 0
for(i in 2:nVisitType) {
sDispersionVisitType[i] ~ dnorm(0, 2^-2)
}
for (i in 1:length(Fish)) {
log(eDensity[i]) <- bDensity + bDensitySite[Site[i]] + bDensityAnnual[Annual[i]] + bDensitySiteAnnual[Site[i],Annual[i]]
eAbundance[i] <- eDensity[i] * SiteLength[i]
logit(eEfficiency[i]) <- logit(Efficiency[i]) + bEfficiencyVisitType[VisitType[i]]
log(esDispersion[i]) <- sDispersion + sDispersionVisitType[VisitType[i]]
eDispersion[i] ~ dgamma(esDispersion[i]^-2 + 0.1, esDispersion[i]^-2 + 0.1)
eFish[i] <- eAbundance[i] * ProportionSampled[i] * eEfficiency[i]
Fish[i] ~ dpois(eFish[i] * eDispersion[i])
}
..
Block 6.
Stock-Recruitment
.model {
bAlpha ~ dnorm(1, 2^-2) T(log(1), log(5))
bBeta ~ dnorm(-10, 5^-2)
bEggLoss ~ dnorm(0, 2^-2)
sRecruits ~ dnorm(0, 1^-2) T(0,)
for(i in 1:length(Stock)){
log(eAlpha[i]) <- bAlpha
log(eBeta[i]) <- bBeta
eLogRecruits[i] <- log((eAlpha[i] * Stock[i]) / (1 + eBeta[i] * Stock[i])) + bEggLoss * EggLoss[i]
Recruits[i] ~ dlnorm(eLogRecruits[i], sRecruits^-2)
}
..
Block 7.
Age-Ratios
.model{
bProbAge1 ~ dnorm(0, 2^-2)
bProbAge1Loss ~ dnorm(0, 2^-2)
sProbAge1 ~ dunif(0, 2)
for(i in 1:length(Age1Prop)){
eAge1Prop[i] <- bProbAge1 + bProbAge1Loss * LossLogRatio[i]
Age1Prop[i] ~ dnorm(eAge1Prop[i], sProbAge1^-2)
}
..
Block 8.
Results
Tables
Condition
Table 1. Parameter descriptions.
Parameter | Description |
---|---|
bWeight |
Intercept of log(eWeight) |
bWeightDayte |
Effect of Dayte on bWeight |
bWeightLength |
Intercept of effect of Length on bWeight |
bWeightLengthDayte |
Effect of Dayte on bWeightLength |
bWeightLengthYear[i] |
Effect of i th Year on bWeightLength |
bWeightYear[i] |
Effect of i th Year on bWeight |
Dayte[i] |
Standardised day of year i th fish was captured |
eWeight[i] |
Expected Weight of i th fish |
Length[i] |
Log-transformed and centered fork length of i th fish |
sWeight |
Log standard deviation of residual variation in log(Weight) |
sWeightLengthYear |
Log standard deviation of bWeightLengthYear |
sWeightYear |
Log standard deviation of bWeightYear |
Weight[i] |
Recorded weight of i th fish |
Year[i] |
Year i th fish was captured |
Mountain Whitefish
Table 2. Model coefficients.
term | estimate | sd | zscore | lower | upper | pvalue |
---|---|---|---|---|---|---|
bWeight | 5.4481708 | 0.0099010 | 550.217864 | 5.4277038 | 5.4674031 | 0.0007 |
bWeightDayte | -0.0176867 | 0.0018633 | -9.517441 | -0.0213734 | -0.0141445 | 0.0007 |
bWeightLength | 3.1579282 | 0.0233766 | 135.080079 | 3.1093590 | 3.2040653 | 0.0007 |
bWeightLengthDayte | -0.0122878 | 0.0050029 | -2.457204 | -0.0221361 | -0.0026500 | 0.0093 |
sWeight | -1.9095091 | 0.0060218 | -317.076006 | -1.9208347 | -1.8977600 | 0.0007 |
sWeightLengthYear | -2.2657732 | 0.1913599 | -11.805313 | -2.6206595 | -1.8801177 | 0.0007 |
sWeightYear | -3.1215164 | 0.1734585 | -17.942536 | -3.4339287 | -2.7488788 | 0.0007 |
Table 3. Model summary.
n | K | nchains | niters | nthin | ess | rhat | converged |
---|---|---|---|---|---|---|---|
14178 | 7 | 3 | 500 | 2 | 393 | 1.011 | TRUE |
Rainbow Trout
Table 4. Model coefficients.
term | estimate | sd | zscore | lower | upper | pvalue |
---|---|---|---|---|---|---|
bWeight | 5.9948771 | 0.0062049 | 966.160917 | 5.9819110 | 6.0072682 | 7e-04 |
bWeightDayte | -0.0044249 | 0.0012834 | -3.475668 | -0.0069787 | -0.0019914 | 7e-04 |
bWeightLength | 2.9215460 | 0.0125398 | 232.960088 | 2.8962627 | 2.9453204 | 7e-04 |
bWeightLengthDayte | 0.0377059 | 0.0038700 | 9.744408 | 0.0301796 | 0.0451479 | 7e-04 |
sWeight | -2.2655650 | 0.0058125 | -389.785952 | -2.2774300 | -2.2545811 | 7e-04 |
sWeightLengthYear | -2.9060607 | 0.1892851 | -15.342687 | -3.2594914 | -2.5229922 | 7e-04 |
sWeightYear | -3.6317633 | 0.1684327 | -21.507633 | -3.9415447 | -3.2894396 | 7e-04 |
Table 5. Model summary.
n | K | nchains | niters | nthin | ess | rhat | converged |
---|---|---|---|---|---|---|---|
14589 | 7 | 3 | 500 | 2 | 374 | 1.009 | TRUE |
Walleye
Table 6. Model coefficients.
term | estimate | sd | zscore | lower | upper | pvalue |
---|---|---|---|---|---|---|
bWeight | 6.2919286 | 0.0080132 | 785.139922 | 6.2739150 | 6.3064459 | 0.0007 |
bWeightDayte | 0.0161593 | 0.0014148 | 11.389367 | 0.0134151 | 0.0188725 | 0.0007 |
bWeightLength | 3.2304215 | 0.0191397 | 168.779806 | 3.1904598 | 3.2675943 | 0.0007 |
bWeightLengthDayte | -0.0107375 | 0.0083310 | -1.277545 | -0.0272052 | 0.0058224 | 0.1947 |
sWeight | -2.3681131 | 0.0073079 | -324.012600 | -2.3819666 | -2.3535583 | 0.0007 |
sWeightLengthYear | -2.5323101 | 0.1992700 | -12.680344 | -2.8975376 | -2.1164227 | 0.0007 |
sWeightYear | -3.3305458 | 0.1668623 | -19.908489 | -3.6302314 | -2.9748845 | 0.0007 |
Table 7. Model summary.
n | K | nchains | niters | nthin | ess | rhat | converged |
---|---|---|---|---|---|---|---|
9205 | 7 | 3 | 500 | 2 | 393 | 1.014 | TRUE |
Growth
Table 8. Parameter descriptions.
Parameter | Description |
---|---|
bK |
Intercept of log(eK) |
bKYear[i] |
Effect of i th Year on bK |
bLinf |
Mean maximum length |
dYears[i] |
Years between release and recapture of i th recapture |
eGrowth |
Expected Growth between release and recapture |
eK[i] |
Expected von Bertalanffy growth coefficient from i-1 th to i th year |
Growth[i] |
Observed growth between release and recapture of i th recapture |
LengthAtRelease[i] |
Length at previous release of i th recapture |
sGrowth |
Log standard deviation of residual variation in Growth |
sKYear |
Log standard deviation of bKYear |
Year[i] |
Release year of i th recapture |
Mountain Whitefish
Table 9. Model coefficients.
term | estimate | sd | zscore | lower | upper | pvalue |
---|---|---|---|---|---|---|
bK | -0.9259595 | 0.1086078 | -8.559326 | -1.149029 | -0.7297757 | 7e-04 |
bLinf | 392.6903086 | 3.2148232 | 122.202222 | 386.888094 | 399.3993119 | 7e-04 |
sGrowth | 2.4601904 | 0.0443263 | 55.511488 | 2.377194 | 2.5493541 | 7e-04 |
sKYear | -1.0848023 | 0.2570075 | -4.222173 | -1.557970 | -0.5837363 | 7e-04 |
Table 10. Model summary.
n | K | nchains | niters | nthin | ess | rhat | converged |
---|---|---|---|---|---|---|---|
259 | 4 | 3 | 500 | 20 | 543 | 1.013 | TRUE |
Rainbow Trout
Table 11. Model coefficients.
term | estimate | sd | zscore | lower | upper | pvalue |
---|---|---|---|---|---|---|
bK | -0.1744708 | 0.0762919 | -2.306990 | -0.3307308 | -0.0225079 | 2e-02 |
bLinf | 487.0687845 | 2.7075093 | 179.890601 | 481.9086684 | 492.4080715 | 7e-04 |
sGrowth | 3.3811271 | 0.0206856 | 163.459867 | 3.3411191 | 3.4218987 | 7e-04 |
sKYear | -1.2416744 | 0.1924957 | -6.405807 | -1.5677043 | -0.8305064 | 7e-04 |
Table 12. Model summary.
n | K | nchains | niters | nthin | ess | rhat | converged |
---|---|---|---|---|---|---|---|
1158 | 4 | 3 | 500 | 20 | 400 | 1.011 | TRUE |
Walleye
Table 13. Model coefficients.
term | estimate | sd | zscore | lower | upper | pvalue |
---|---|---|---|---|---|---|
bK | -2.539502 | 0.2502549 | -10.147884 | -3.001751 | -2.0618755 | 7e-04 |
bLinf | 754.315450 | 84.3008026 | 9.083864 | 628.631688 | 949.4953241 | 7e-04 |
sGrowth | 2.866226 | 0.0460119 | 62.295651 | 2.778109 | 2.9608715 | 7e-04 |
sKYear | -1.157805 | 0.2545813 | -4.530802 | -1.636968 | -0.6447953 | 7e-04 |
Table 14. Model summary.
n | K | nchains | niters | nthin | ess | rhat | converged |
---|---|---|---|---|---|---|---|
263 | 4 | 3 | 500 | 40 | 222 | 1.006 | TRUE |
Movement
Table 15. Parameter descriptions.
Parameter | Description |
---|---|
bFidelity |
Intercept of logit(eFidelity) |
bLength |
Effect of length on logit(eFidelity) |
eFidelity[i] |
Expected site fidelity of i th recapture |
Fidelity[i] |
Whether the i th recapture was encountered at the same
site as the previous encounter |
Length[i] |
Length at previous encounter of i th recapture |
Mountain Whitefish
Table 16. Model coefficients.
term | estimate | sd | zscore | lower | upper | pvalue |
---|---|---|---|---|---|---|
bFidelity | -0.1552880 | 0.1792936 | -0.8567948 | -0.4959377 | 0.1981948 | 0.388 |
bLength | -0.1105809 | 0.1946158 | -0.5319073 | -0.4760564 | 0.2944789 | 0.592 |
Table 17. Model summary.
n | K | nchains | niters | nthin | ess | rhat | converged |
---|---|---|---|---|---|---|---|
117 | 2 | 3 | 500 | 1 | 847 | 1.002 | TRUE |
Rainbow Trout
Table 18. Model coefficients.
term | estimate | sd | zscore | lower | upper | pvalue |
---|---|---|---|---|---|---|
bFidelity | 0.7654122 | 0.0796299 | 9.626364 | 0.6143531 | 0.9178480 | 7e-04 |
bLength | -0.3300007 | 0.0769163 | -4.272205 | -0.4739996 | -0.1766411 | 7e-04 |
Table 19. Model summary.
n | K | nchains | niters | nthin | ess | rhat | converged |
---|---|---|---|---|---|---|---|
756 | 2 | 3 | 500 | 1 | 774 | 1.001 | TRUE |
Walleye
Table 20. Model coefficients.
term | estimate | sd | zscore | lower | upper | pvalue |
---|---|---|---|---|---|---|
bFidelity | 0.7258183 | 0.1486066 | 4.9023664 | 0.4342957 | 1.0092428 | 0.0007 |
bLength | -0.0188939 | 0.1390122 | -0.1504418 | -0.2987952 | 0.2483826 | 0.8800 |
Table 21. Model summary.
n | K | nchains | niters | nthin | ess | rhat | converged |
---|---|---|---|---|---|---|---|
220 | 2 | 3 | 500 | 1 | 942 | 1.003 | TRUE |
Length-At-Age
Mountain Whitefish
Table 22. The estimated upper length cutoffs (mm) by age and year.
Year | Age0 | Age1 | Age2 |
---|---|---|---|
1990 | 163 | 275 | NA |
1991 | 144 | 226 | 296 |
2001 | 141 | 257 | 344 |
2002 | 163 | 260 | 343 |
2003 | 159 | 263 | 353 |
2004 | 158 | 249 | 342 |
2005 | 168 | 263 | 362 |
2006 | 175 | 284 | 357 |
2007 | 171 | 279 | 337 |
2008 | 170 | 248 | 341 |
2009 | 169 | 265 | 355 |
2010 | 177 | 272 | 353 |
2011 | 163 | 269 | 349 |
2012 | 162 | 268 | 347 |
2013 | 185 | 282 | 350 |
2014 | 178 | 283 | 362 |
2015 | 167 | 278 | 366 |
2016 | 165 | 283 | 352 |
2017 | 158 | 269 | 354 |
2018 | 177 | 262 | 346 |
Rainbow Trout
Table 23. The estimated upper length cutoffs (mm) by age and year.
Year | Age0 | Age1 |
---|---|---|
1990 | 155 | 358 |
1991 | 127 | 342 |
2001 | 133 | 324 |
2002 | 155 | 349 |
2003 | 161 | 342 |
2004 | 142 | 332 |
2005 | 164 | 346 |
2006 | 170 | 364 |
2007 | 166 | 375 |
2008 | 146 | 339 |
2009 | 147 | 338 |
2010 | 143 | 337 |
2011 | 156 | 343 |
2012 | 152 | 344 |
2013 | 169 | 354 |
2014 | 154 | 337 |
2015 | 167 | 334 |
2016 | 154 | 336 |
2017 | 133 | 316 |
2018 | 139 | 306 |
Survival
Table 24. Parameter descriptions.
Parameter | Description |
---|---|
bEfficiency |
Intercept for logit(eEfficiency) |
bEfficiencySampledLength |
Effect of SampledLength on bEfficiency |
bSurvival |
Intercept for logit(eSurvival) |
bSurvivalYear[i] |
Effect of Year on bSurvival |
eEfficiency[i] |
Expected recapture probability in i th year |
eSurvival[i] |
Expected survival probability from i-1 th to i th year |
SampledLength |
Total standardised length of river sampled |
sSurvivalYear |
Log SD of bSurvivalYear |
Mountain Whitefish
Table 25. Model coefficients.
term | estimate | sd | zscore | lower | upper | pvalue |
---|---|---|---|---|---|---|
bEfficiency | -4.1804013 | 0.1042621 | -40.087541 | -4.3845229 | -3.9700036 | 0.0007 |
bEfficiencySampledLength | 0.3721012 | 0.1235324 | 3.044773 | 0.1361591 | 0.6260196 | 0.0027 |
bSurvival | 0.8463913 | 0.4231537 | 2.100816 | 0.1262261 | 1.8068358 | 0.0253 |
sSurvivalYear | 0.2442526 | 0.3452415 | 0.739245 | -0.4118839 | 0.9193125 | 0.4587 |
Table 26. Model summary.
n | K | nchains | niters | nthin | ess | rhat | converged |
---|---|---|---|---|---|---|---|
17 | 4 | 3 | 500 | 100 | 1052 | 1.005 | TRUE |
Rainbow Trout
Table 27. Model coefficients.
term | estimate | sd | zscore | lower | upper | pvalue |
---|---|---|---|---|---|---|
bEfficiency | -2.5293162 | 0.0910345 | -27.7736502 | -2.7044300 | -2.3480069 | 0.0007 |
bEfficiencySampledLength | 0.0111727 | 0.0715640 | 0.1401614 | -0.1288650 | 0.1565815 | 0.8907 |
bSurvival | -0.4348034 | 0.1118249 | -3.8860616 | -0.6559384 | -0.2061208 | 0.0007 |
sSurvivalYear | -1.2570805 | 1.0270840 | -1.4226367 | -4.9922516 | -0.6119388 | 0.0007 |
Table 28. Model summary.
n | K | nchains | niters | nthin | ess | rhat | converged |
---|---|---|---|---|---|---|---|
17 | 4 | 3 | 500 | 100 | 261 | 1.017 | TRUE |
Walleye
Table 29. Model coefficients.
term | estimate | sd | zscore | lower | upper | pvalue |
---|---|---|---|---|---|---|
bEfficiency | -3.3757879 | 0.1030395 | -32.8019436 | -3.5811971 | -3.1708020 | 0.0007 |
bEfficiencySampledLength | 0.0587105 | 0.0826296 | 0.7621854 | -0.0929374 | 0.2316543 | 0.4440 |
bSurvival | 0.0628850 | 0.1358752 | 0.5602158 | -0.1683843 | 0.3885211 | 0.5440 |
sSurvivalYear | -1.0997005 | 1.9896128 | -0.9160875 | -8.2747079 | -0.2785067 | 0.0040 |
Table 30. Model summary.
n | K | nchains | niters | nthin | ess | rhat | converged |
---|---|---|---|---|---|---|---|
17 | 4 | 3 | 500 | 200 | 285 | 1.012 | TRUE |
Capture Efficiency
Table 31. Parameter descriptions.
Parameter | Description |
---|---|
Annual[i] |
Year of i th visit |
bEfficiency |
Intercept for logit(eEfficiency) |
bEfficiencySessionAnnual |
Effect of Session within Annual on
logit(eEfficiency) |
eEfficiency[i] |
Expected efficiency on i th visit |
eFidelity[i] |
Expected site fidelity on i th visit |
Fidelity[i] |
Mean site fidelity on i th visit |
FidelitySD[i] |
SD of site fidelity on i th visit |
Recaptures[i] |
Number of marked fish recaught during i th visit |
sEfficiencySessionAnnual |
Log SD of effect of Session within Annual on
logit(eEfficiency) |
Session[i] |
Session of i th visit |
Tagged[i] |
Number of marked fish tagged prior to i th visit |
Mountain Whitefish
Subadult
Table 32. Model coefficients.
term | estimate | sd | zscore | lower | upper | pvalue |
---|---|---|---|---|---|---|
bEfficiency | -4.9972025 | 0.2168087 | -23.144160 | -5.50063 | -4.6570936 | 0.0007 |
sEfficiencySessionAnnual | -0.9461967 | 1.1534489 | -1.110064 | -4.39114 | 0.0966403 | 0.0907 |
Table 33. Model summary.
n | K | nchains | niters | nthin | ess | rhat | converged |
---|---|---|---|---|---|---|---|
1359 | 2 | 3 | 500 | 100 | 172 | 1.011 | TRUE |
Adult
Table 34. Model coefficients.
term | estimate | sd | zscore | lower | upper | pvalue |
---|---|---|---|---|---|---|
bEfficiency | -5.254932 | 0.1430929 | -36.755286 | -5.550742 | -4.9969882 | 7e-04 |
sEfficiencySessionAnnual | -2.049795 | 1.1473121 | -1.939951 | -5.029096 | -0.5983976 | 7e-04 |
Table 35. Model summary.
n | K | nchains | niters | nthin | ess | rhat | converged |
---|---|---|---|---|---|---|---|
1545 | 2 | 3 | 500 | 200 | 356 | 1.01 | TRUE |
Rainbow Trout
Subadult
Table 36. Model coefficients.
term | estimate | sd | zscore | lower | upper | pvalue |
---|---|---|---|---|---|---|
bEfficiency | -3.3923810 | 0.0654085 | -51.880249 | -3.524317 | -3.2695877 | 7e-04 |
sEfficiencySessionAnnual | -0.9259046 | 0.1647731 | -5.624789 | -1.263903 | -0.6153031 | 7e-04 |
Table 37. Model summary.
n | K | nchains | niters | nthin | ess | rhat | converged |
---|---|---|---|---|---|---|---|
1558 | 2 | 3 | 500 | 100 | 1214 | 1.005 | TRUE |
Adult
Table 38. Model coefficients.
term | estimate | sd | zscore | lower | upper | pvalue |
---|---|---|---|---|---|---|
bEfficiency | -4.019309 | 0.0710880 | -56.58399 | -4.162507 | -3.8854350 | 7e-04 |
sEfficiencySessionAnnual | -1.805271 | 0.8881719 | -2.28053 | -4.395410 | -0.9157114 | 7e-04 |
Table 39. Model summary.
n | K | nchains | niters | nthin | ess | rhat | converged |
---|---|---|---|---|---|---|---|
1629 | 2 | 3 | 500 | 100 | 244 | 1.009 | TRUE |
Walleye
Table 40. Model coefficients.
term | estimate | sd | zscore | lower | upper | pvalue |
---|---|---|---|---|---|---|
bEfficiency | -4.5702137 | 0.1218943 | -37.523799 | -4.8186384 | -4.3526356 | 0.0007 |
sEfficiencySessionAnnual | -0.5577893 | 0.2079586 | -2.704928 | -0.9992889 | -0.1707303 | 0.0013 |
Table 41. Model summary.
n | K | nchains | niters | nthin | ess | rhat | converged |
---|---|---|---|---|---|---|---|
1673 | 2 | 3 | 500 | 100 | 1191 | 1.004 | TRUE |
Abundance
Table 42. Parameter descriptions.
Parameter | Description |
---|---|
Annual |
Year |
bDensity |
Intercept for log(eDensity) |
bDensityAnnual |
Effect of Annual on bDensity |
bDensitySite |
Effect of Site on bDensity |
bDensitySiteAnnual |
Effect of Site within Annual on bDensity |
bEfficiencyVisitType |
Effect of VisitType on Efficiency |
eDensity |
Expected density |
Efficiency |
Capture efficiency |
esDispersion |
Overdispersion of Fish |
Fish |
Number of fish captured or counted |
ProportionSampled |
Proportion of site surveyed |
sDensityAnnual |
Log SD of effect of Annual on bDensity |
sDensitySite |
Log SD of effect of Site on bDensity |
sDensitySiteAnnual |
Log SD of effect of Site within Annual on bDensity |
sDispersion |
Intercept for log(esDispersion) |
sDispersionVisitType |
Effect of VisitType on sDispersion |
Site |
Site |
SiteLength |
Length of site |
VisitType |
Survey type (catch versus count) |
Mountain Whitefish
Subadult
Table 43. Model coefficients.
term | estimate | sd | zscore | lower | upper | pvalue |
---|---|---|---|---|---|---|
bDensity | 5.4406580 | 0.1874181 | 29.013274 | 5.0405099 | 5.7930340 | 0.0007 |
bEfficiencyVisitType[2] | 1.4556995 | 0.0806910 | 18.040699 | 1.2955386 | 1.6214112 | 0.0007 |
sDensityAnnual | -0.3775397 | 0.1736743 | -2.125087 | -0.6879389 | -0.0297508 | 0.0387 |
sDensitySite | -0.2804839 | 0.1087247 | -2.544630 | -0.4863189 | -0.0637620 | 0.0120 |
sDensitySiteAnnual | -0.8244691 | 0.0611086 | -13.458192 | -0.9419095 | -0.7030915 | 0.0007 |
sDispersion | -0.7661634 | 0.0449215 | -17.069776 | -0.8547301 | -0.6842493 | 0.0007 |
sDispersionVisitType[2] | 0.6067664 | 0.0927844 | 6.551051 | 0.4258833 | 0.7808791 | 0.0007 |
Table 44. Model summary.
n | K | nchains | niters | nthin | ess | rhat | converged |
---|---|---|---|---|---|---|---|
2551 | 7 | 3 | 500 | 200 | 242 | 1.009 | TRUE |
Adult
Table 45. Model coefficients.
term | estimate | sd | zscore | lower | upper | pvalue |
---|---|---|---|---|---|---|
bDensity | 6.3926287 | 0.1626779 | 39.271714 | 6.0770985 | 6.7111804 | 0.0007 |
bEfficiencyVisitType[2] | 1.7286939 | 0.0804946 | 21.492578 | 1.5693144 | 1.8887179 | 0.0007 |
sDensityAnnual | -1.0772117 | 0.1991107 | -5.379547 | -1.4486766 | -0.6795237 | 0.0007 |
sDensitySite | 0.1034875 | 0.0951208 | 1.059008 | -0.0796085 | 0.2881605 | 0.2853 |
sDensitySiteAnnual | -0.9089343 | 0.0653335 | -13.926549 | -1.0397152 | -0.7879970 | 0.0007 |
sDispersion | -0.6528188 | 0.0346453 | -18.859430 | -0.7171653 | -0.5855589 | 0.0007 |
sDispersionVisitType[2] | 0.4805907 | 0.0803911 | 5.973736 | 0.3199828 | 0.6406288 | 0.0007 |
Table 46. Model summary.
n | K | nchains | niters | nthin | ess | rhat | converged |
---|---|---|---|---|---|---|---|
2551 | 7 | 3 | 500 | 200 | 285 | 1.008 | TRUE |
Rainbow Trout
Subadult
Table 47. Model coefficients.
term | estimate | sd | zscore | lower | upper | pvalue |
---|---|---|---|---|---|---|
bDensity | 4.8366559 | 0.1161441 | 41.651809 | 4.6119463 | 5.0539153 | 7e-04 |
bEfficiencyVisitType[2] | 1.4215737 | 0.0776402 | 18.346412 | 1.2804333 | 1.5847675 | 7e-04 |
sDensityAnnual | -1.1758941 | 0.2012193 | -5.774457 | -1.5226979 | -0.7263475 | 7e-04 |
sDensitySite | -0.3802453 | 0.0972301 | -3.875574 | -0.5617739 | -0.1774587 | 7e-04 |
sDensitySiteAnnual | -0.8930931 | 0.0557025 | -16.052414 | -1.0040788 | -0.7882411 | 7e-04 |
sDispersion | -0.9650807 | 0.0398708 | -24.228399 | -1.0473340 | -0.8914979 | 7e-04 |
sDispersionVisitType[2] | 0.6569666 | 0.0897573 | 7.266721 | 0.4830828 | 0.8315600 | 7e-04 |
Table 48. Model summary.
n | K | nchains | niters | nthin | ess | rhat | converged |
---|---|---|---|---|---|---|---|
2551 | 7 | 3 | 500 | 200 | 494 | 1.008 | TRUE |
Adult
Table 49. Model coefficients.
term | estimate | sd | zscore | lower | upper | pvalue |
---|---|---|---|---|---|---|
bDensity | 5.4942462 | 0.1140338 | 48.164683 | 5.2728280 | 5.7120537 | 7e-04 |
bEfficiencyVisitType[2] | 1.2869357 | 0.0602682 | 21.380955 | 1.1664900 | 1.4055163 | 7e-04 |
sDensityAnnual | -1.0927513 | 0.1848807 | -5.862344 | -1.4319721 | -0.7008315 | 7e-04 |
sDensitySite | -0.4651834 | 0.0931610 | -4.958562 | -0.6378218 | -0.2822007 | 7e-04 |
sDensitySiteAnnual | -1.2089195 | 0.0693396 | -17.453081 | -1.3503149 | -1.0796723 | 7e-04 |
sDispersion | -1.0166974 | 0.0437112 | -23.290154 | -1.1051124 | -0.9356607 | 7e-04 |
sDispersionVisitType[2] | 0.5491976 | 0.0927775 | 5.949737 | 0.3708907 | 0.7335595 | 7e-04 |
Table 50. Model summary.
n | K | nchains | niters | nthin | ess | rhat | converged |
---|---|---|---|---|---|---|---|
2551 | 7 | 3 | 500 | 200 | 538 | 1.006 | TRUE |
Walleye
Table 51. Model coefficients.
term | estimate | sd | zscore | lower | upper | pvalue |
---|---|---|---|---|---|---|
bDensity | 5.4321978 | 0.1158070 | 46.940936 | 5.2043312 | 5.6606843 | 7e-04 |
bEfficiencyVisitType[2] | 1.1735932 | 0.0756155 | 15.543691 | 1.0203961 | 1.3285330 | 7e-04 |
sDensityAnnual | -0.8466866 | 0.1806938 | -4.642299 | -1.1679495 | -0.4744504 | 7e-04 |
sDensitySite | -1.0459901 | 0.1388762 | -7.514605 | -1.3137513 | -0.7765578 | 7e-04 |
sDensitySiteAnnual | -1.3244656 | 0.0846409 | -15.702992 | -1.5117795 | -1.1702710 | 7e-04 |
sDispersion | -0.8224011 | 0.0390031 | -21.050567 | -0.8956316 | -0.7446447 | 7e-04 |
sDispersionVisitType[2] | 0.5073740 | 0.0931255 | 5.432866 | 0.3154596 | 0.6789489 | 7e-04 |
Table 52. Model summary.
n | K | nchains | niters | nthin | ess | rhat | converged |
---|---|---|---|---|---|---|---|
2551 | 7 | 3 | 500 | 200 | 682 | 1.008 | TRUE |
Stock-Recruitment
Table 53. Parameter descriptions.
Parameter | Description |
---|---|
bAlpha |
Intercept for log(eAlpha) |
bBeta |
Intercept for log(eBeta) |
bEggLoss |
Effect of EggLoss on bBeta |
eAlpha |
eRecruits per Stock at low Stock density |
eBeta |
Expected density-dependence |
EggLoss |
Calculated proportional egg loss |
eRecruits |
Expected Recruits |
Recruits |
Number of Age-1 recruits |
sRecruits |
Log SD of residual variation in Recruits |
Stock |
Number of Age-2+ spawners |
Mountain Whitefish
Table 54. Model coefficients.
term | estimate | sd | zscore | lower | upper | pvalue |
---|---|---|---|---|---|---|
bAlpha | 0.9003438 | 0.4528069 | 1.9298312 | 0.0575934 | 1.5750652 | 0.0007 |
bBeta | -9.6517720 | 0.5478618 | -17.7067921 | -10.7832662 | -8.8115021 | 0.0007 |
bEggLoss | -0.1312203 | 0.1693046 | -0.7867717 | -0.4716888 | 0.2092525 | 0.4187 |
sRecruits | 0.6146288 | 0.1334134 | 4.7609870 | 0.4290761 | 0.9387169 | 0.0007 |
Table 55. Model summary.
n | K | nchains | niters | nthin | ess | rhat | converged |
---|---|---|---|---|---|---|---|
16 | 4 | 3 | 500 | 50 | 1262 | 1.002 | TRUE |
Rainbow Trout
Table 56. Model coefficients.
term | estimate | sd | zscore | lower | upper | pvalue |
---|---|---|---|---|---|---|
bAlpha | 1.0563379 | 0.3949465 | 2.567620 | 0.1819702 | 1.5837393 | 0.0007 |
bBeta | -9.1223234 | 0.5321613 | -17.294711 | -10.4142573 | -8.4729122 | 0.0007 |
bEggLoss | 0.1661576 | 0.0742890 | 2.248995 | 0.0252849 | 0.3241453 | 0.0280 |
sRecruits | 0.2746352 | 0.0594218 | 4.793799 | 0.1976847 | 0.4334546 | 0.0007 |
Table 57. Model summary.
n | K | nchains | niters | nthin | ess | rhat | converged |
---|---|---|---|---|---|---|---|
17 | 4 | 3 | 500 | 50 | 532 | 1.006 | TRUE |
Age-Ratios
Table 58. Parameter descriptions.
Parameter | Description |
---|---|
Age1[i] |
The number of Age-1 fish in the i th year |
Age1and2[i] |
The number of Age-1 and Age-2 fish in the i th year |
bProbAge1 |
Intercept for logit(eProbAge1) |
bProbAge1Loss |
Effect of LossLogRatio on bProbAge1 |
eProbAge1[i] |
The expected proportion of Age-1 fish in the i th year |
LossLogRatio[i] |
The log of the ratio of the percent egg losses |
sDispersion |
SD of extra-binomial variation |
Table 59. Model coefficients.
term | estimate | sd | zscore | lower | upper | pvalue |
---|---|---|---|---|---|---|
bProbAge1 | 0.2409373 | 0.2021096 | 1.155502 | -0.1779642 | 0.6160426 | 0.2400 |
bProbAge1Loss | -0.3070399 | 0.2870403 | -1.052766 | -0.8735155 | 0.2271430 | 0.2920 |
sProbAge1 | 0.8203105 | 0.1706548 | 4.943255 | 0.5839656 | 1.2571520 | 0.0007 |
Table 60. Model summary.
n | K | nchains | niters | nthin | ess | rhat | converged |
---|---|---|---|---|---|---|---|
18 | 3 | 3 | 500 | 1 | 668 | 1.006 | TRUE |
Figures
Condition
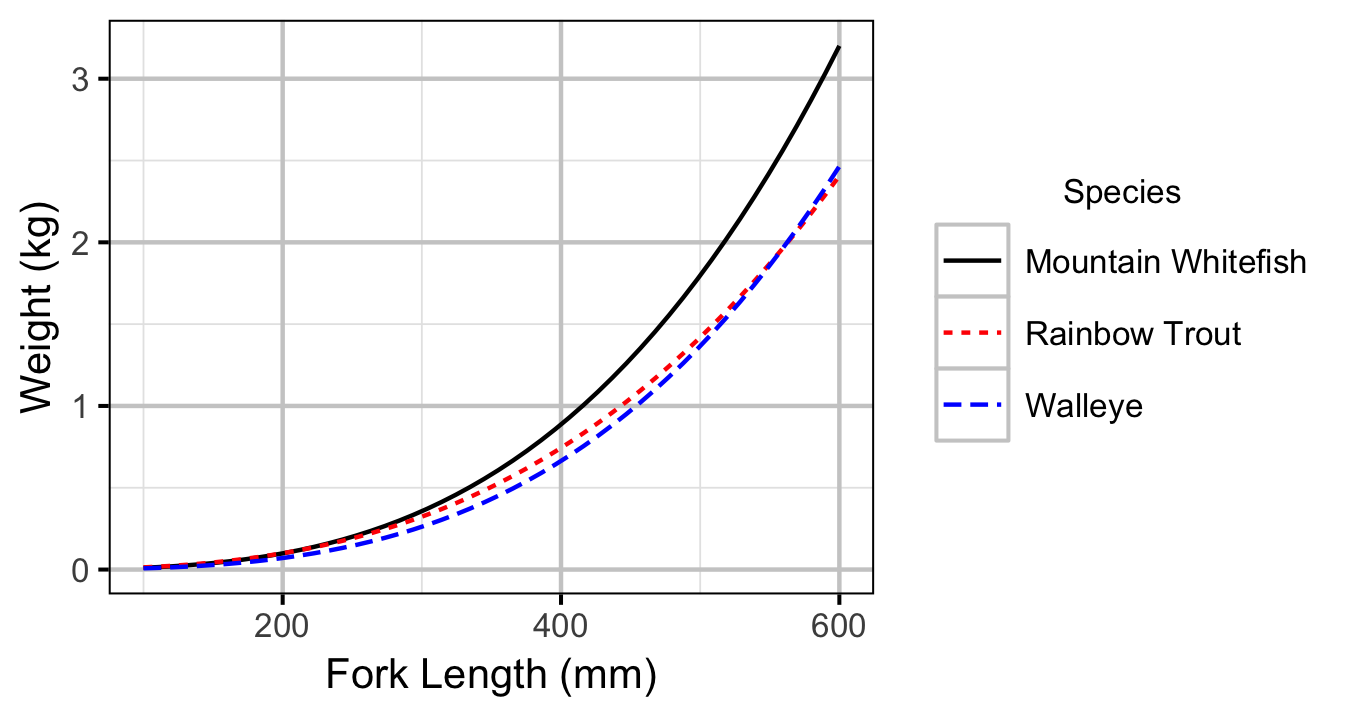
Subadult
Mountain Whitefish
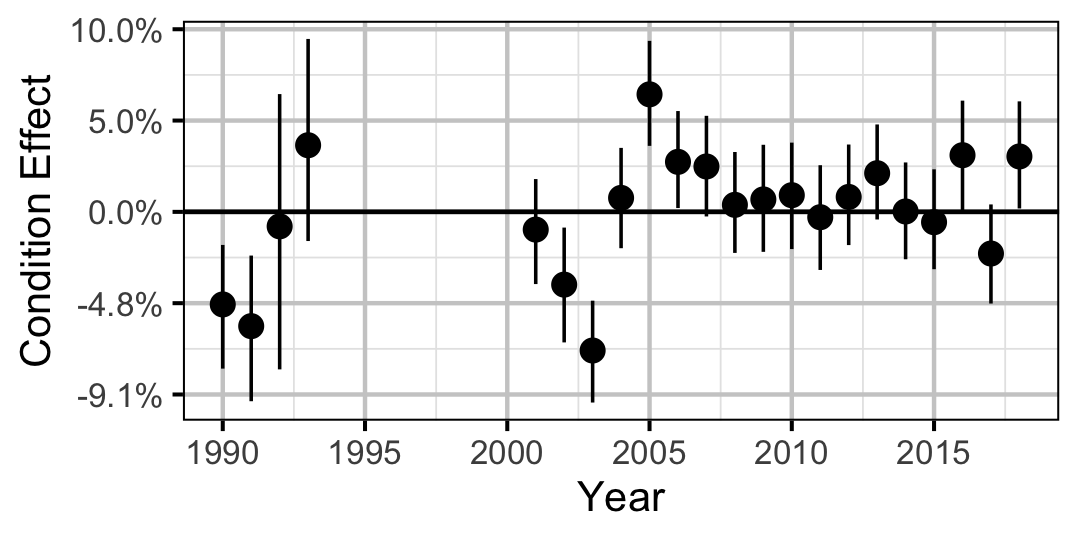
Rainbow Trout
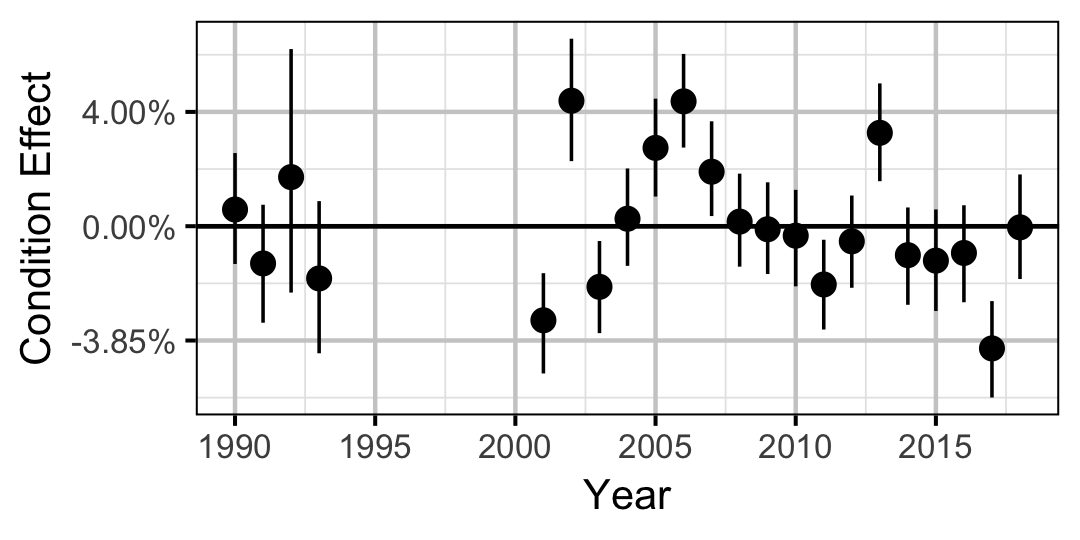
Adult
Mountain Whitefish
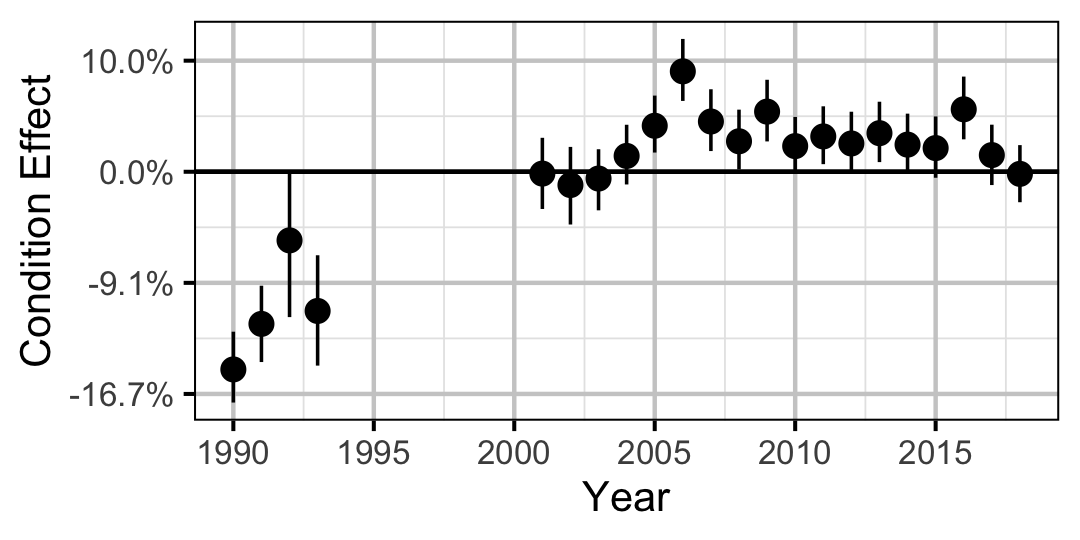
Rainbow Trout
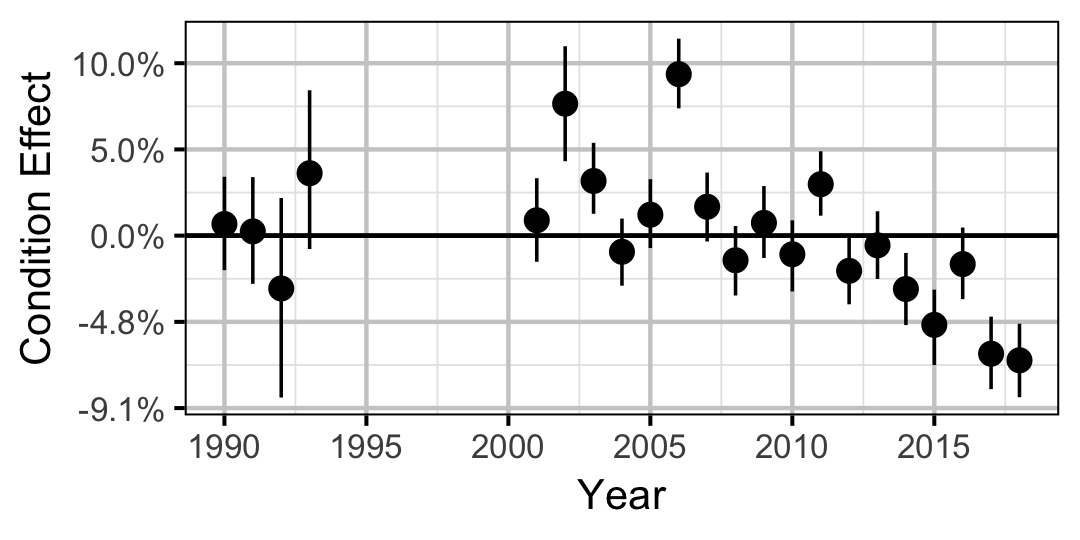
Walleye
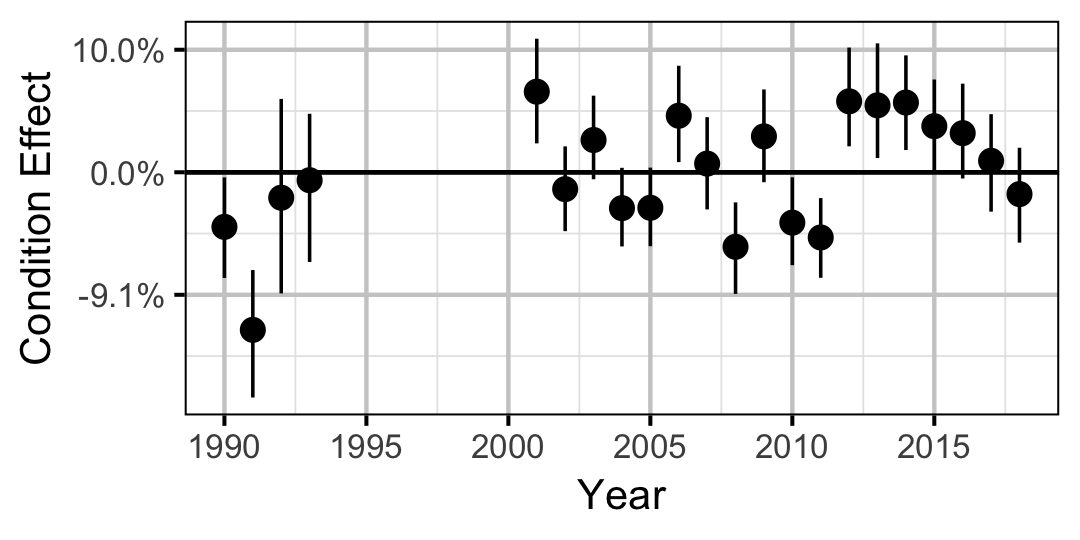
Growth
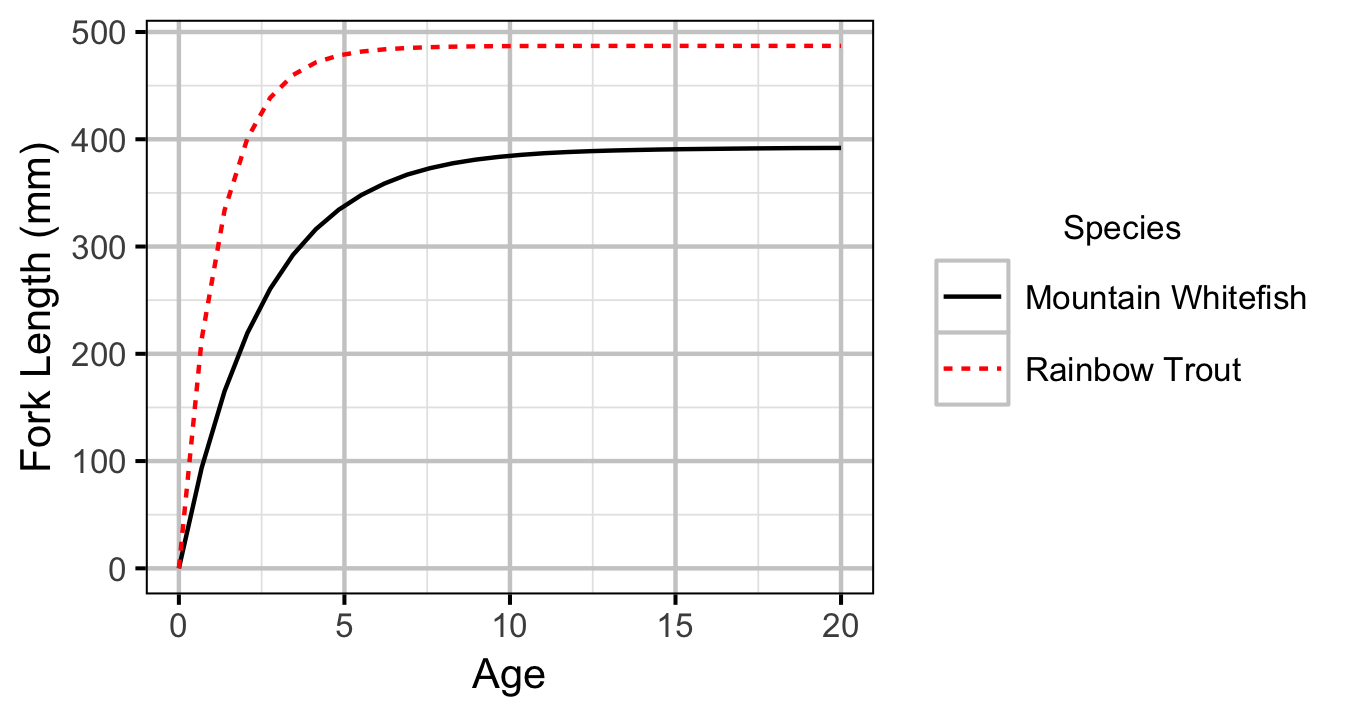
Mountain Whitefish
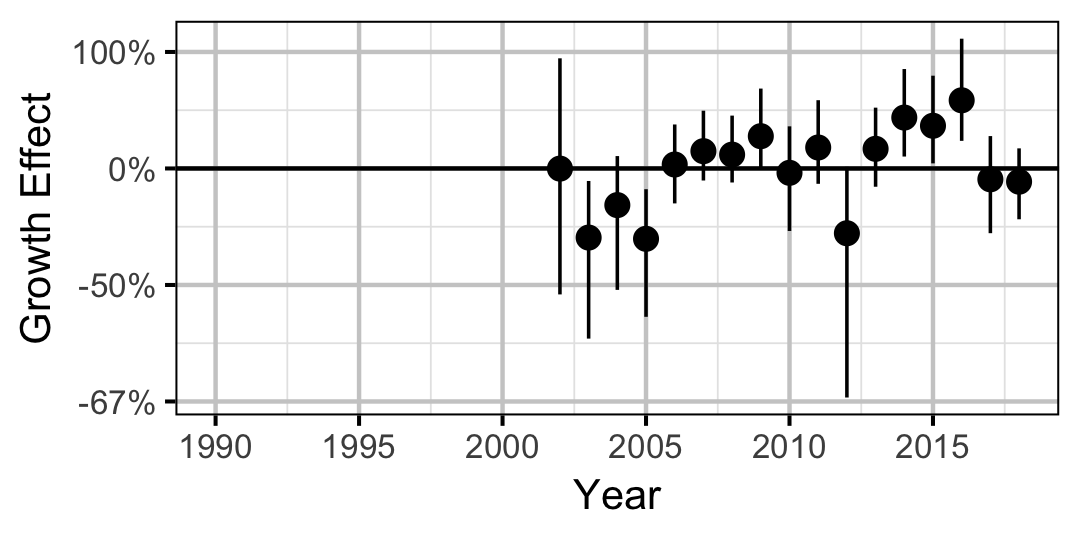
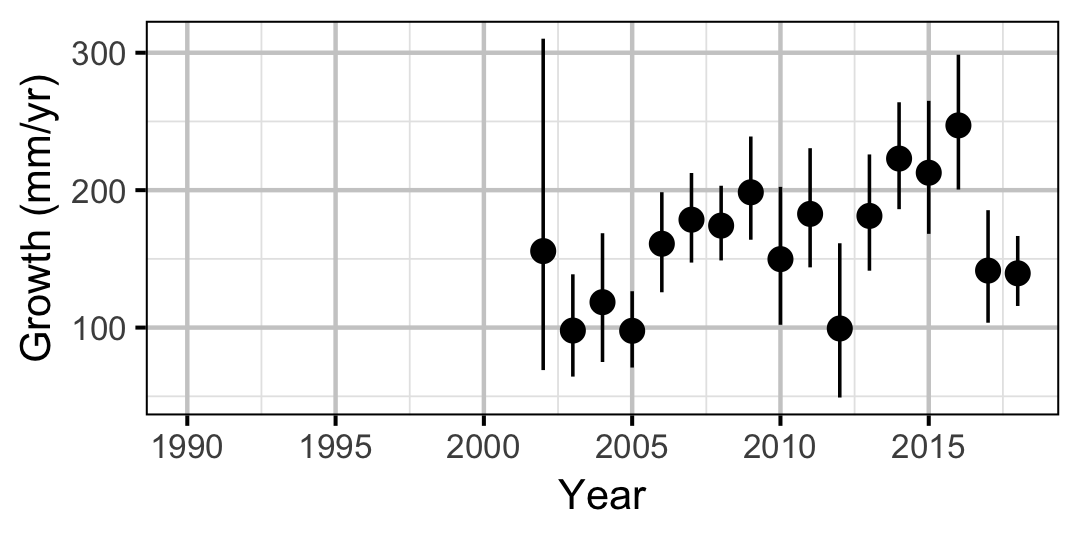
Rainbow Trout
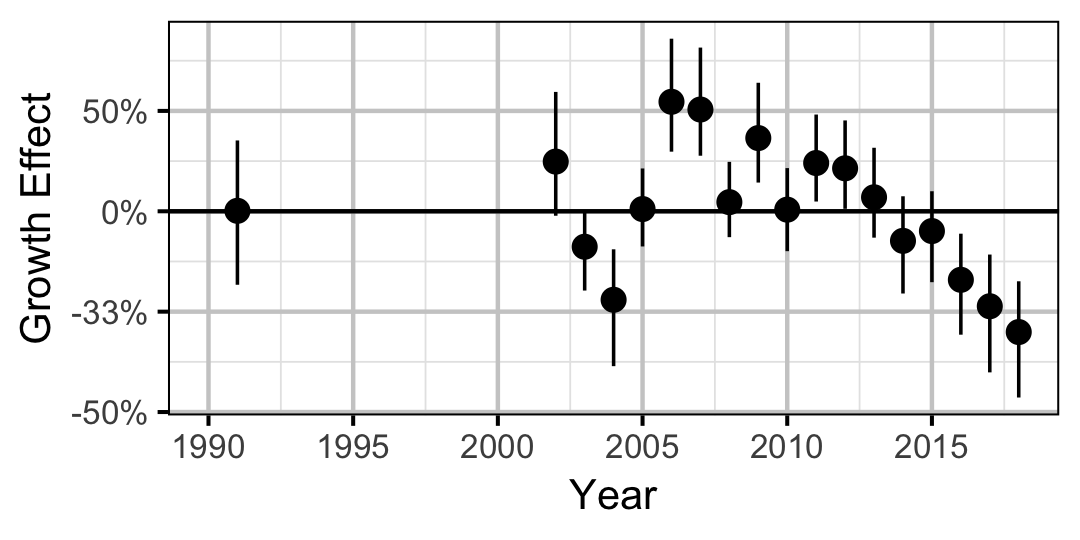
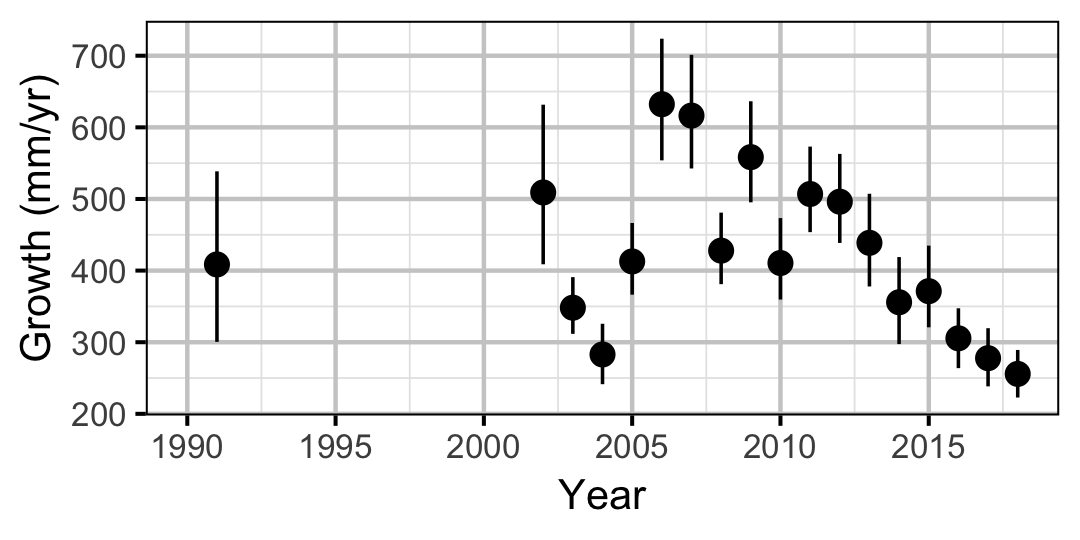
Walleye
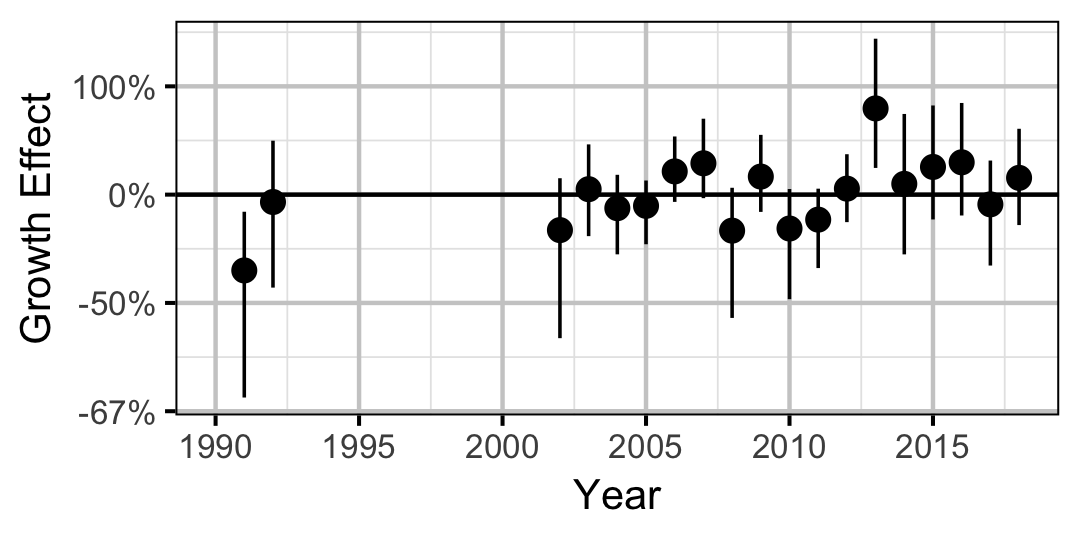
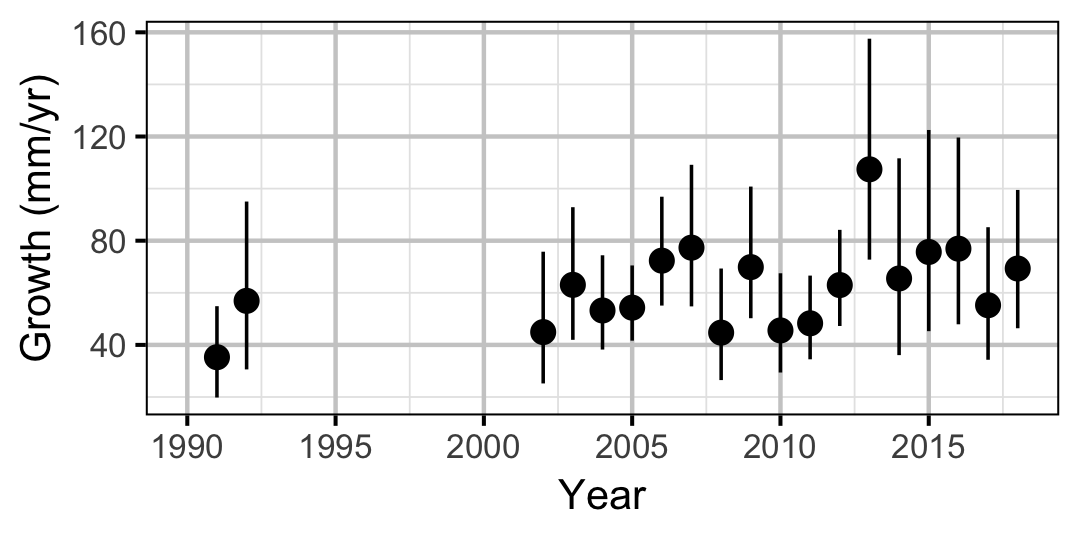
Movement
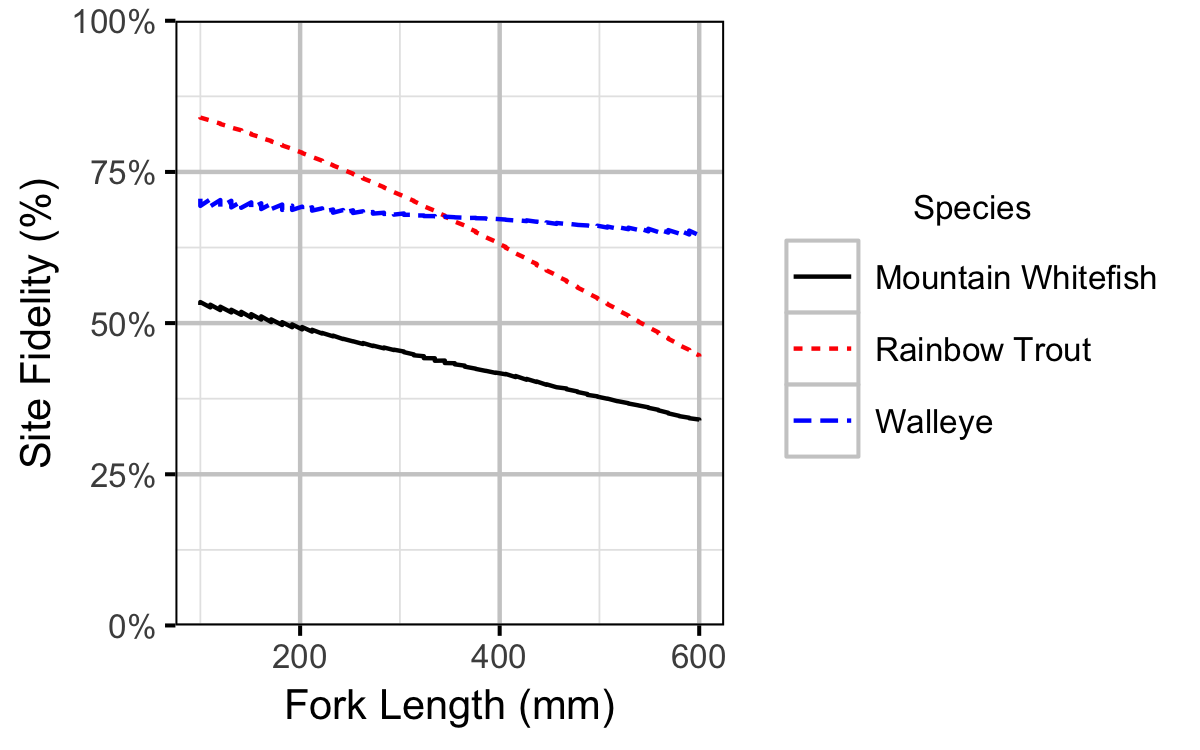
Length-At-Age
Mountain Whitefish
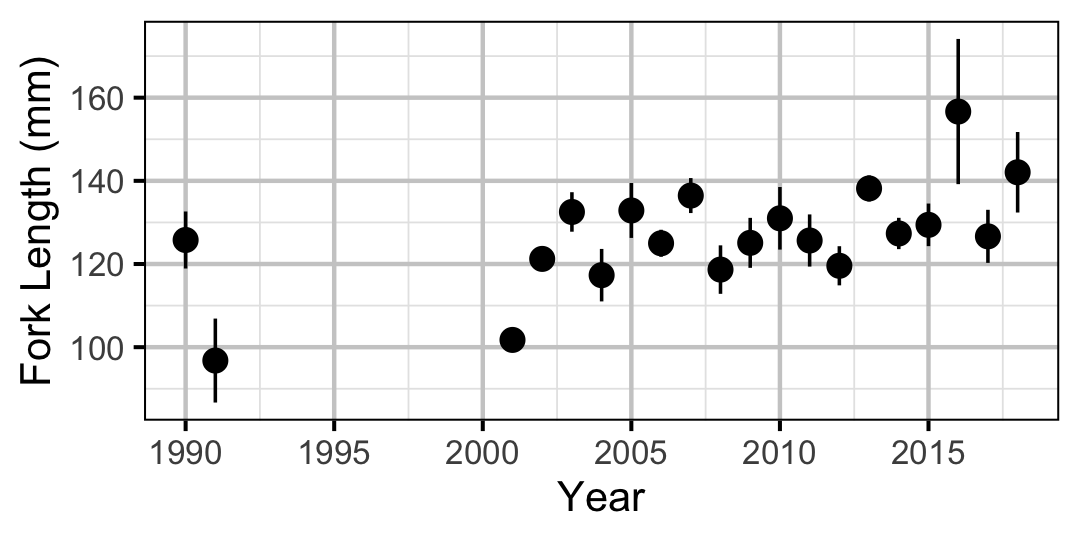
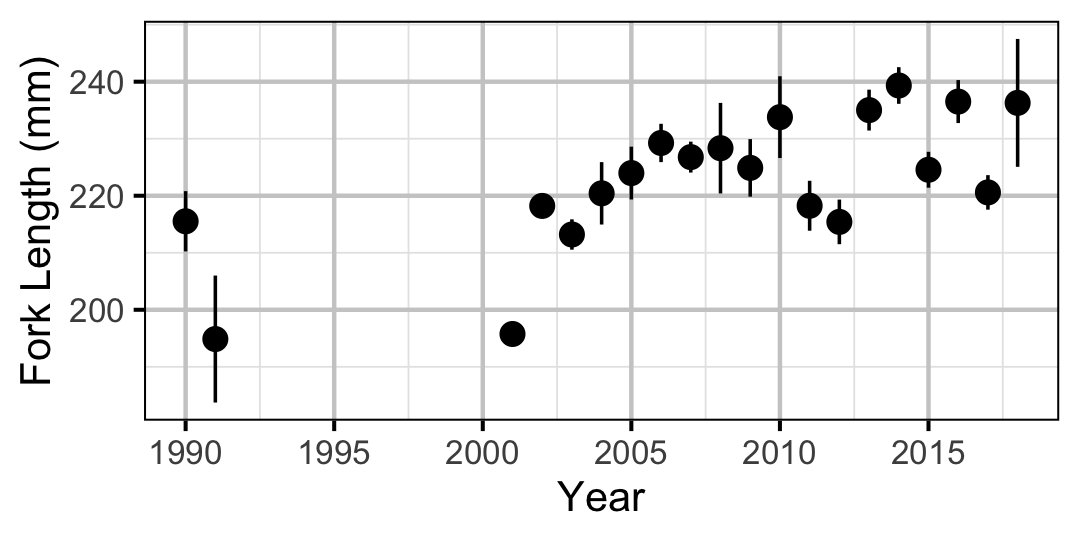
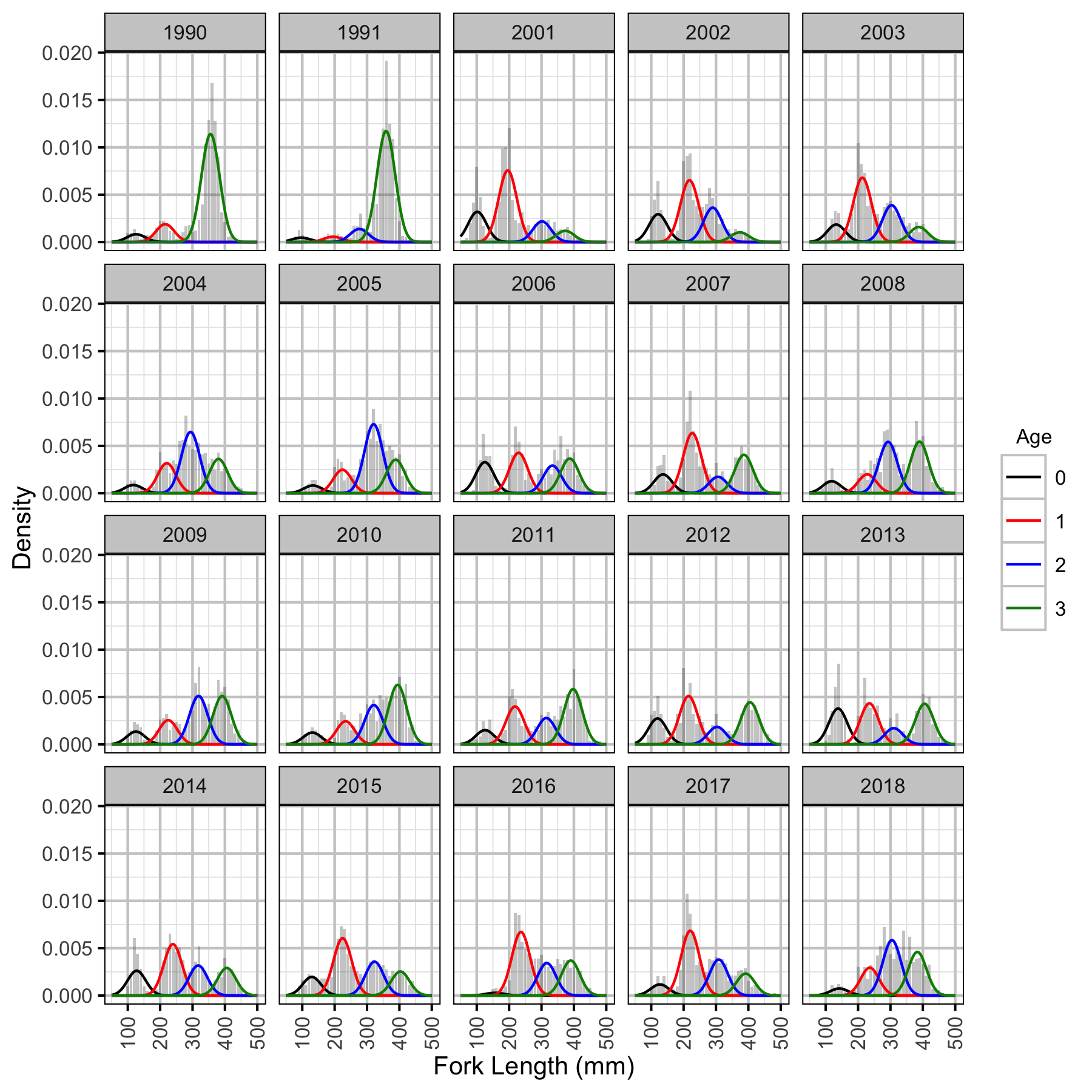
Rainbow Trout
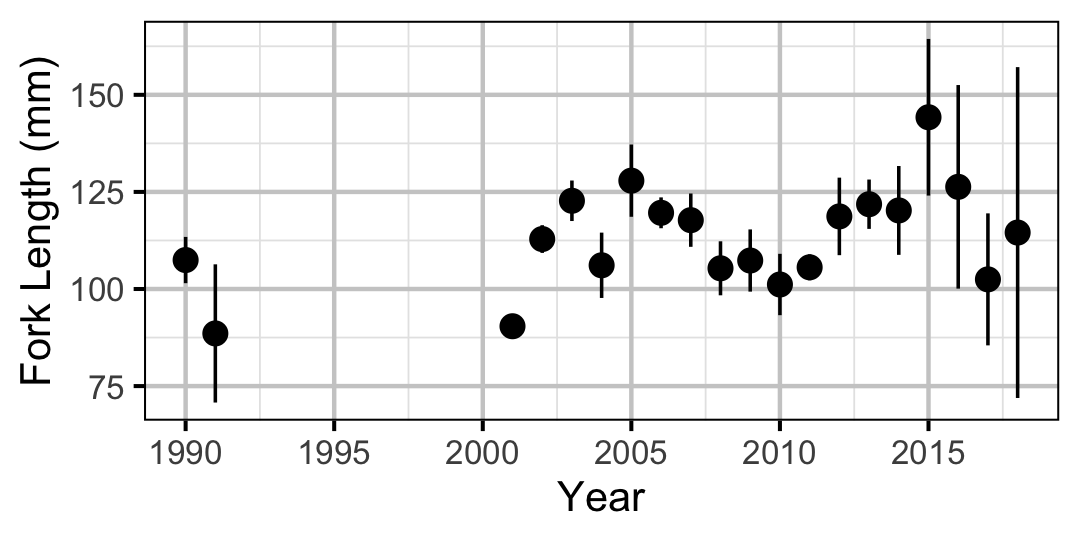
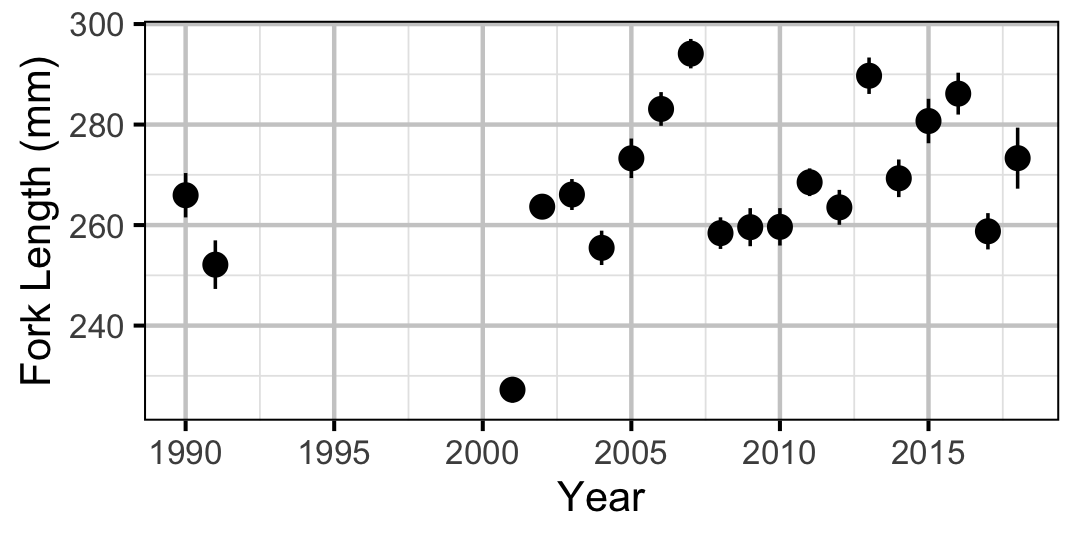
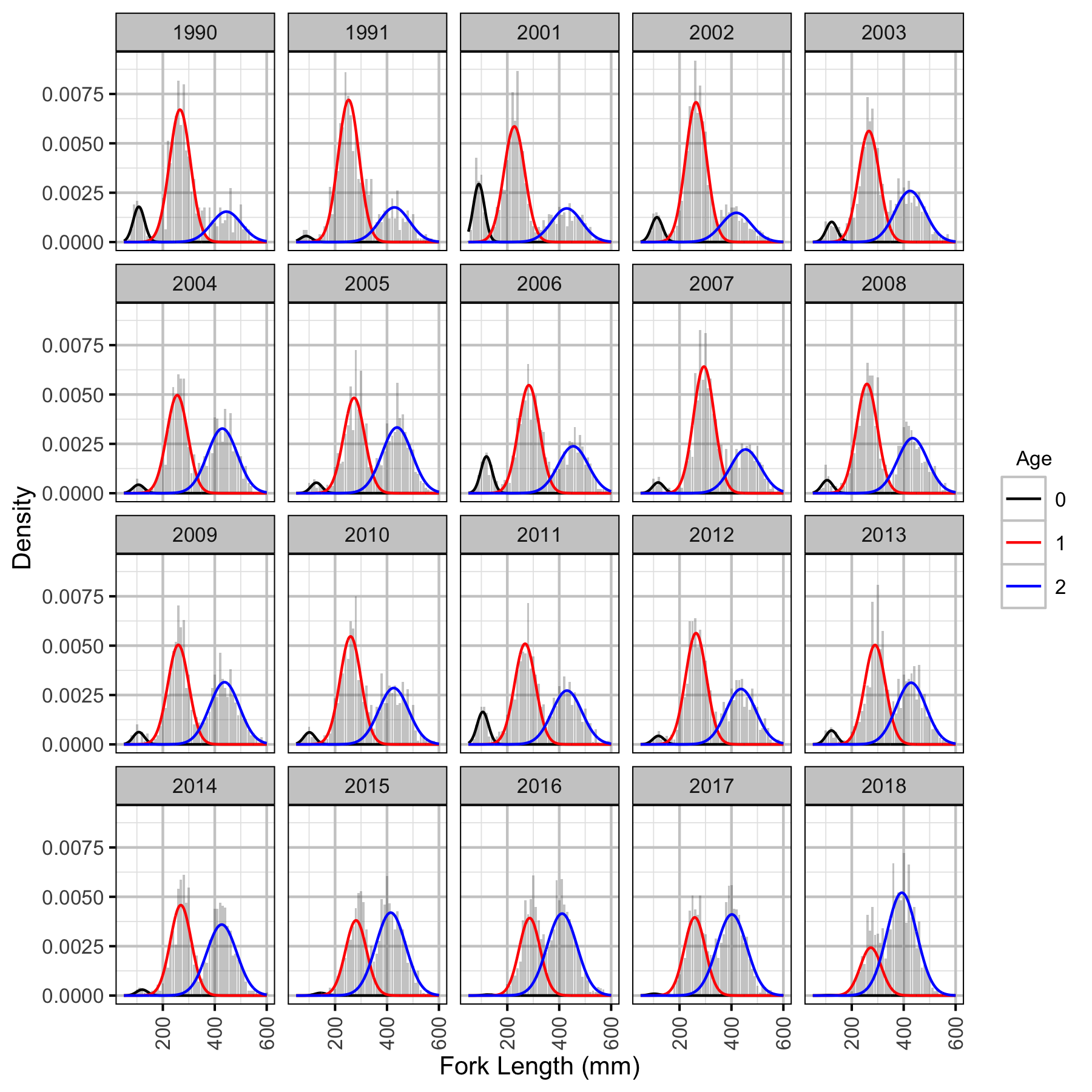
Survival
Adult
Mountain Whitefish
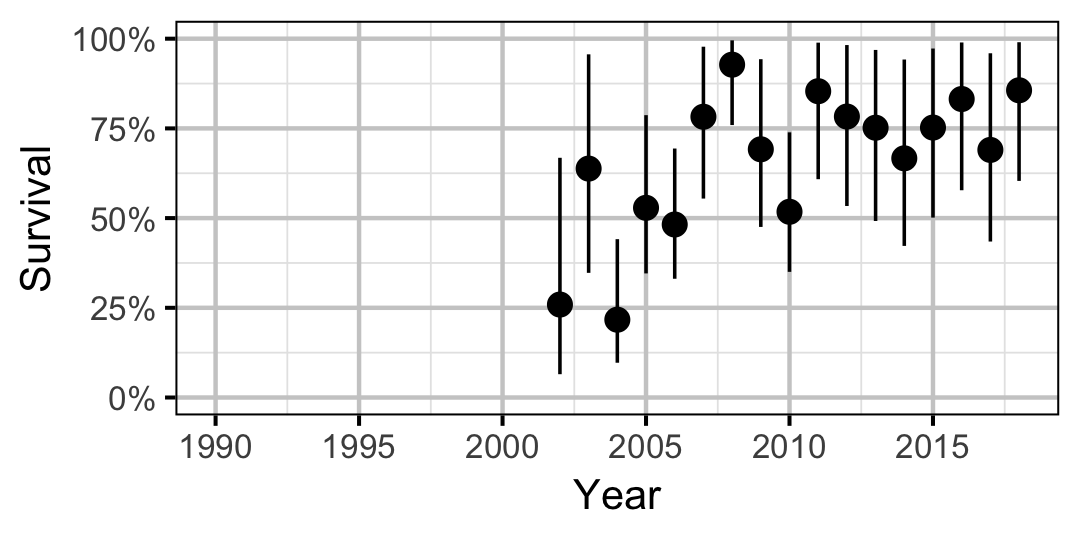
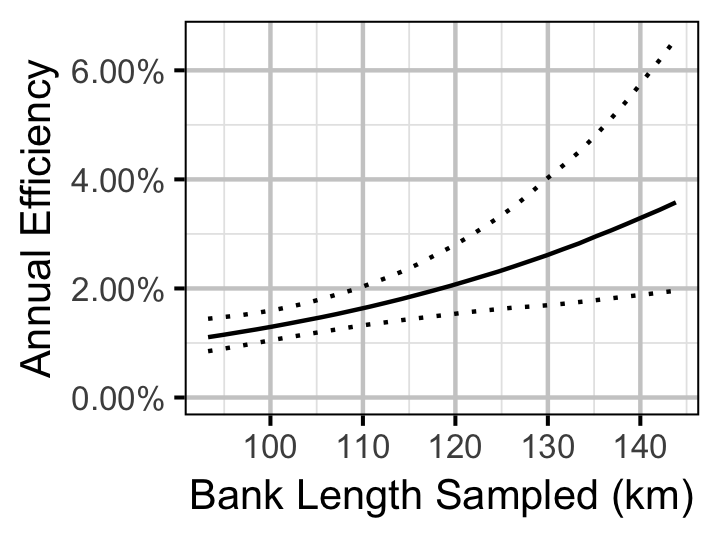
Rainbow Trout
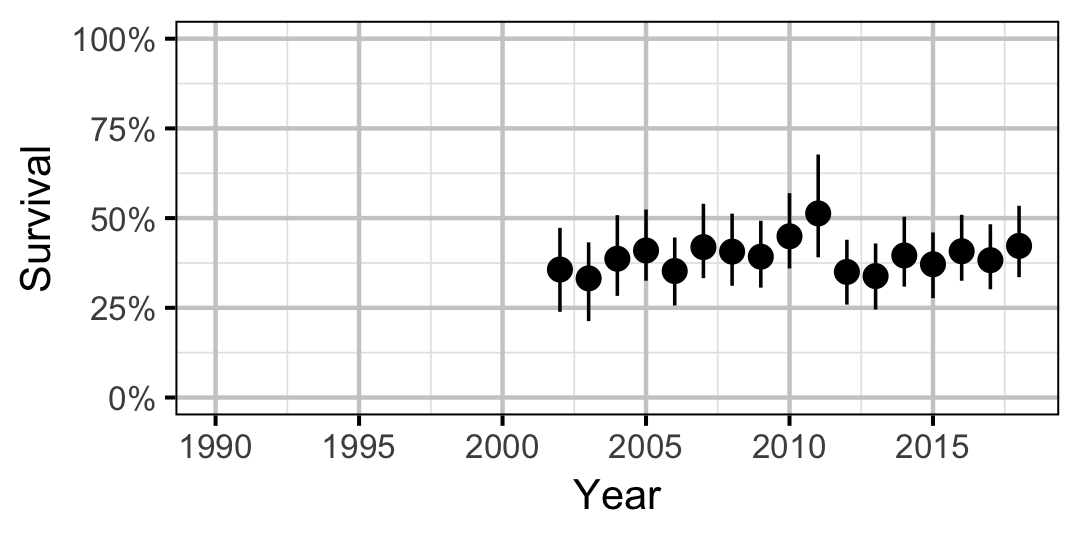
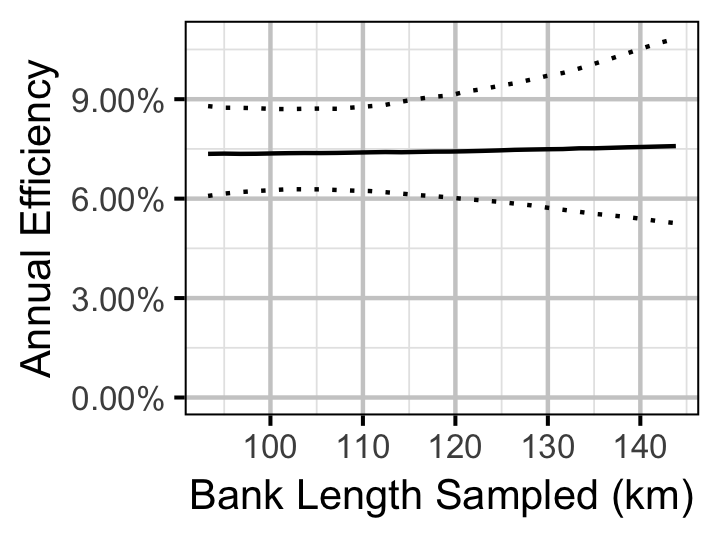
Walleye
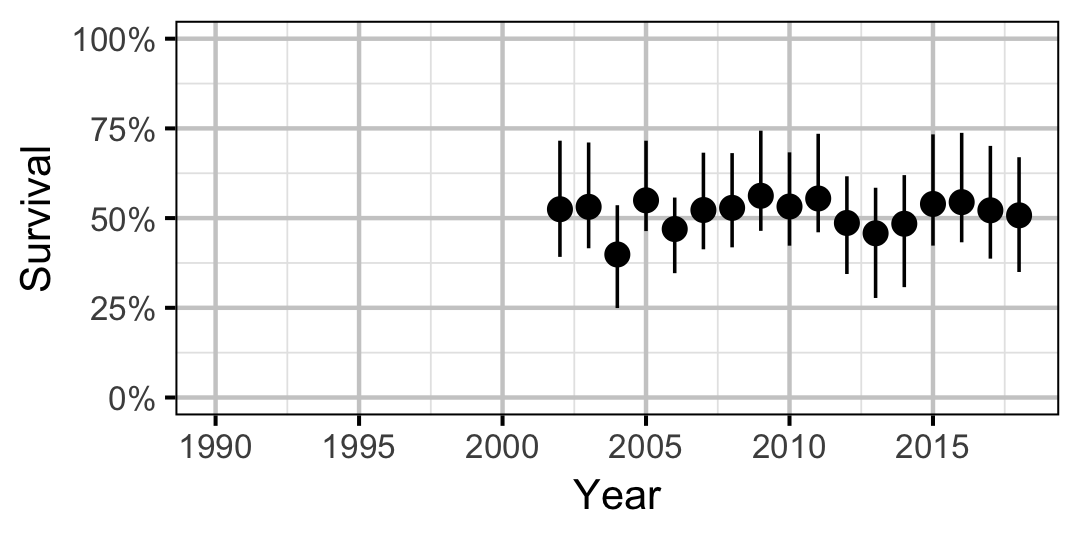
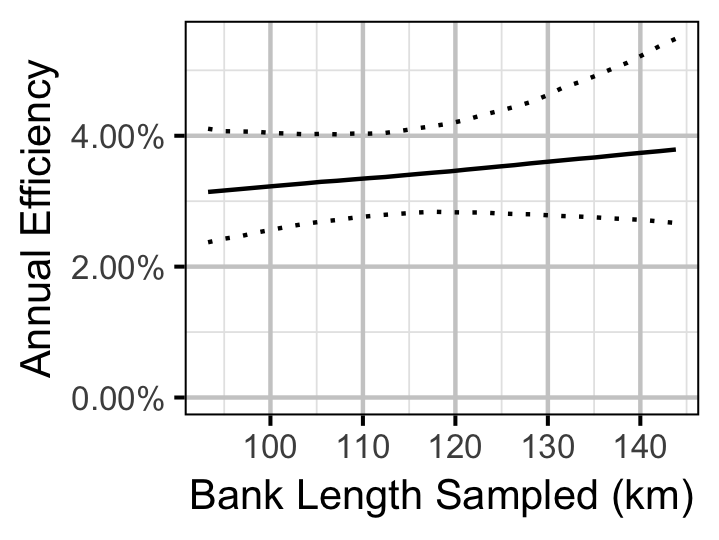
Observer Length Correction
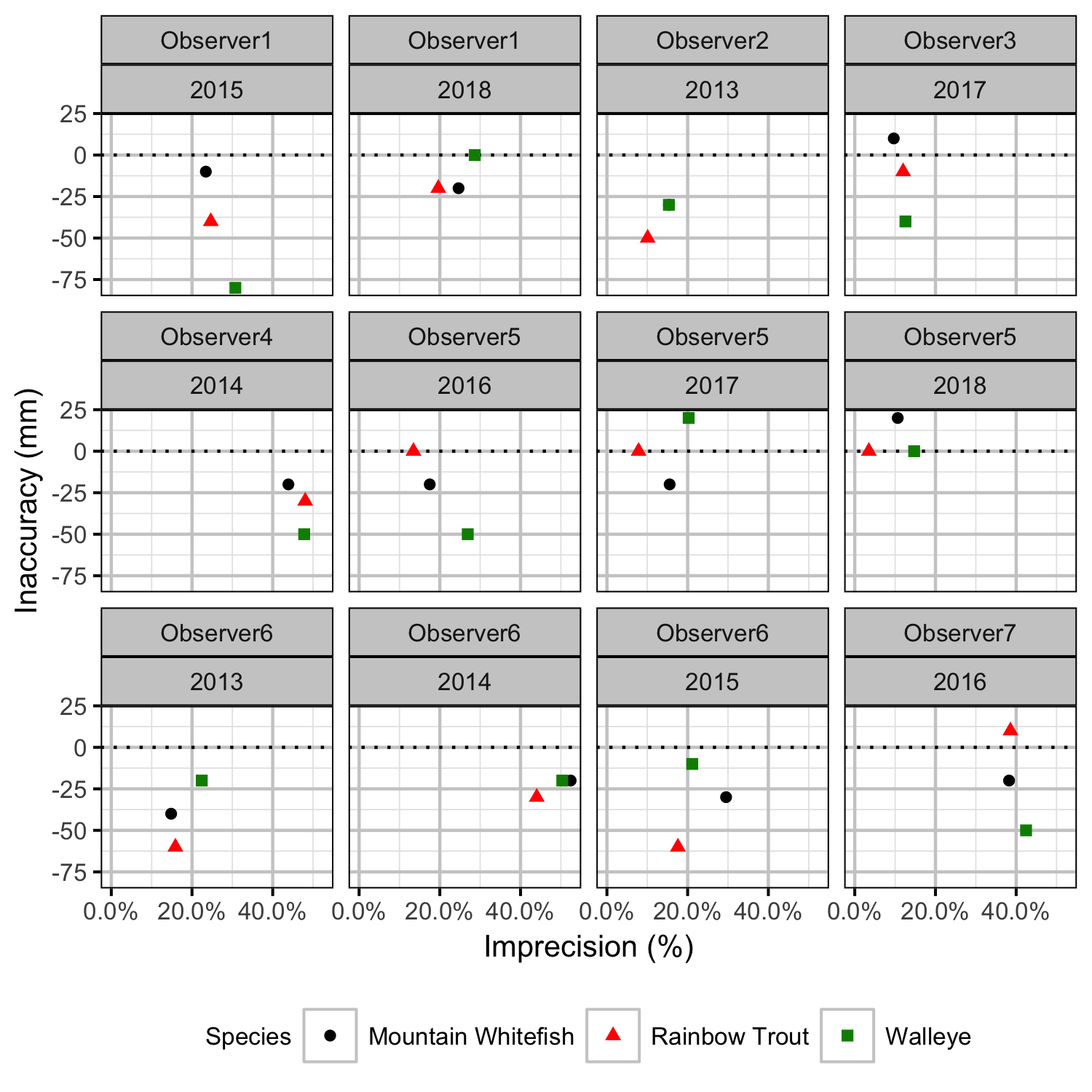
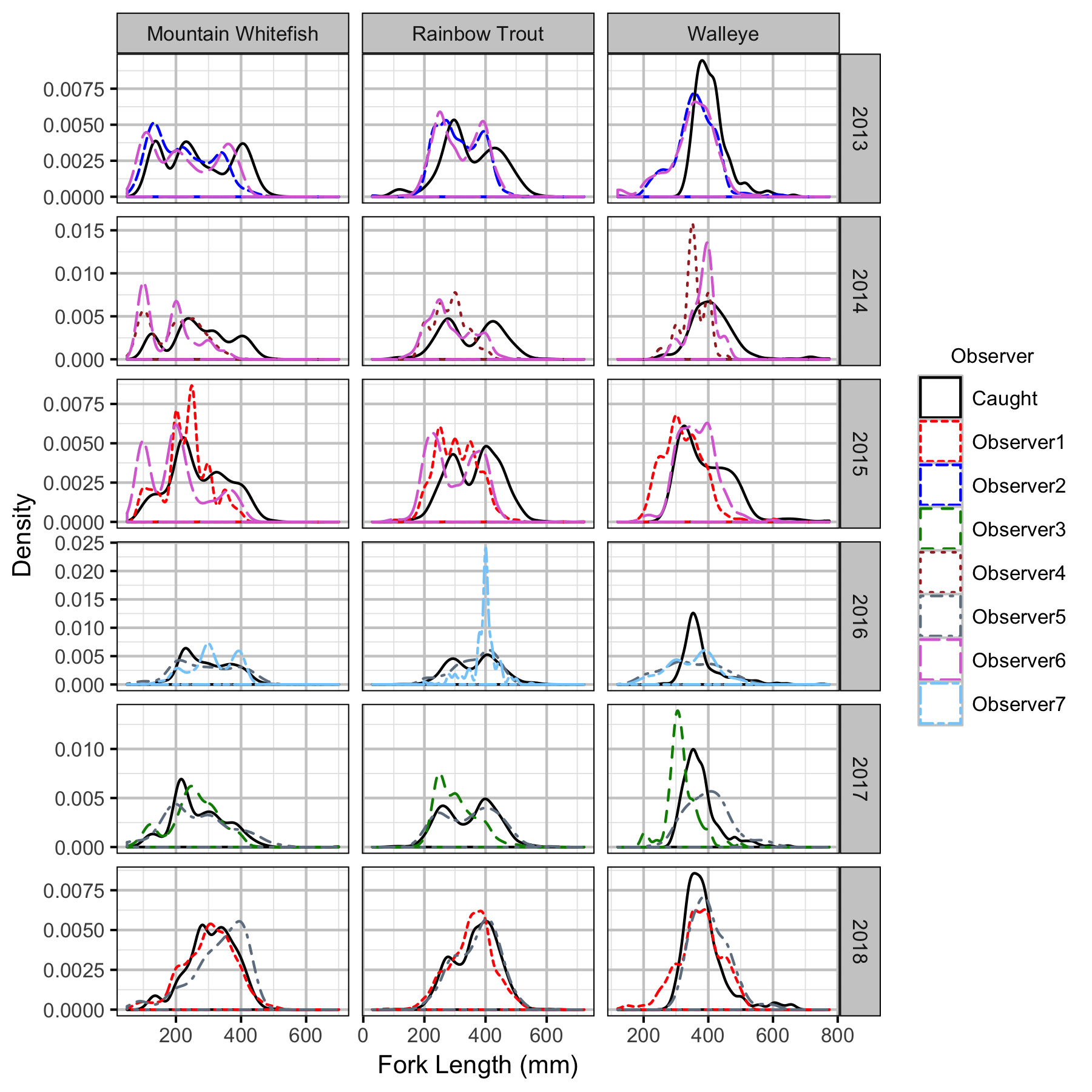
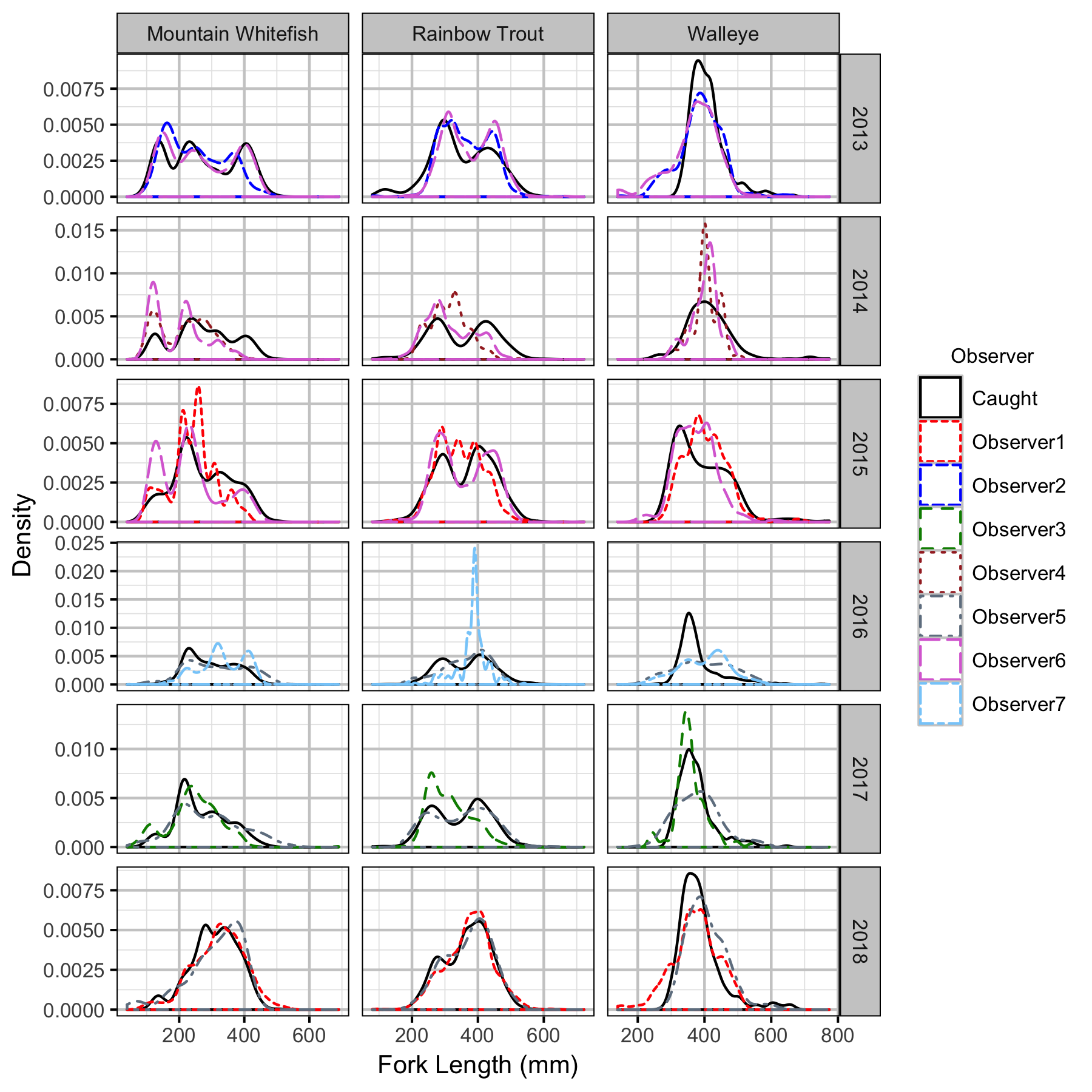
Capture Efficiency
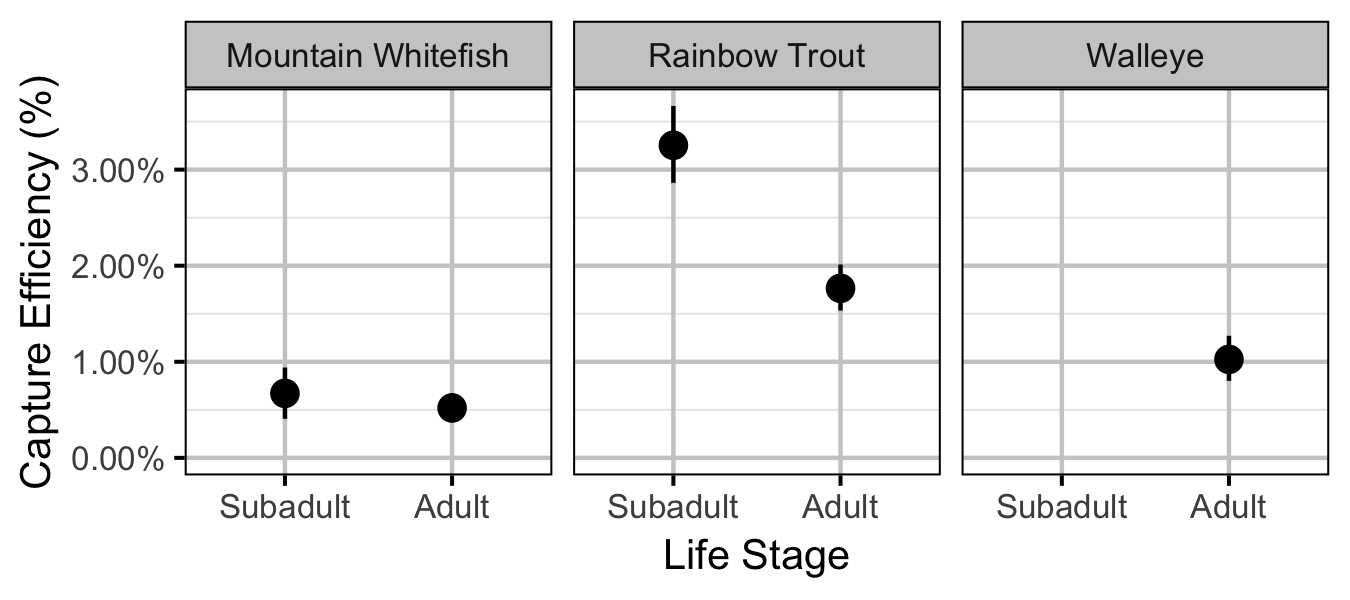
Mountain Whitefish
Subadult
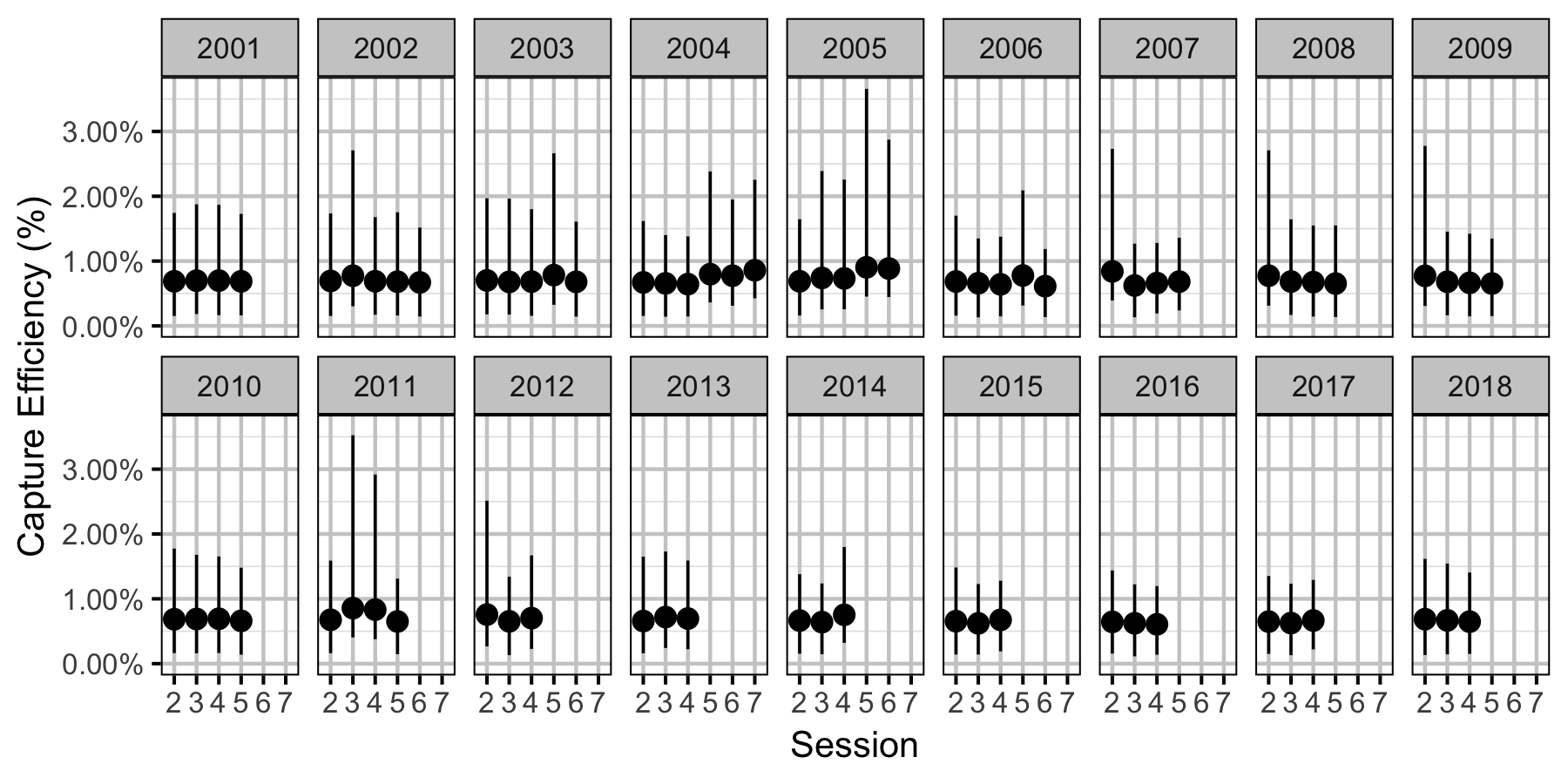
Adult
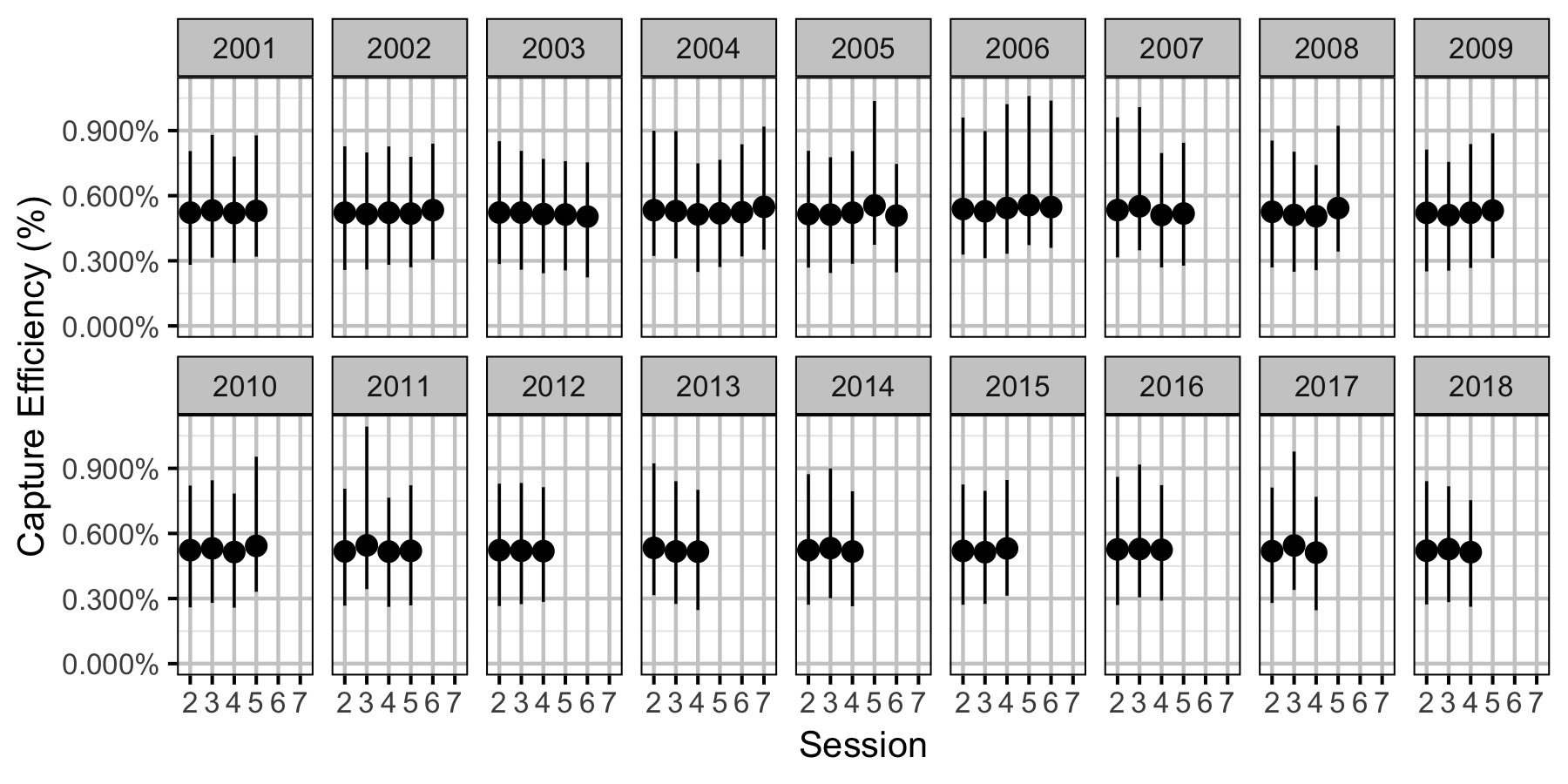
Rainbow Trout
Subadult
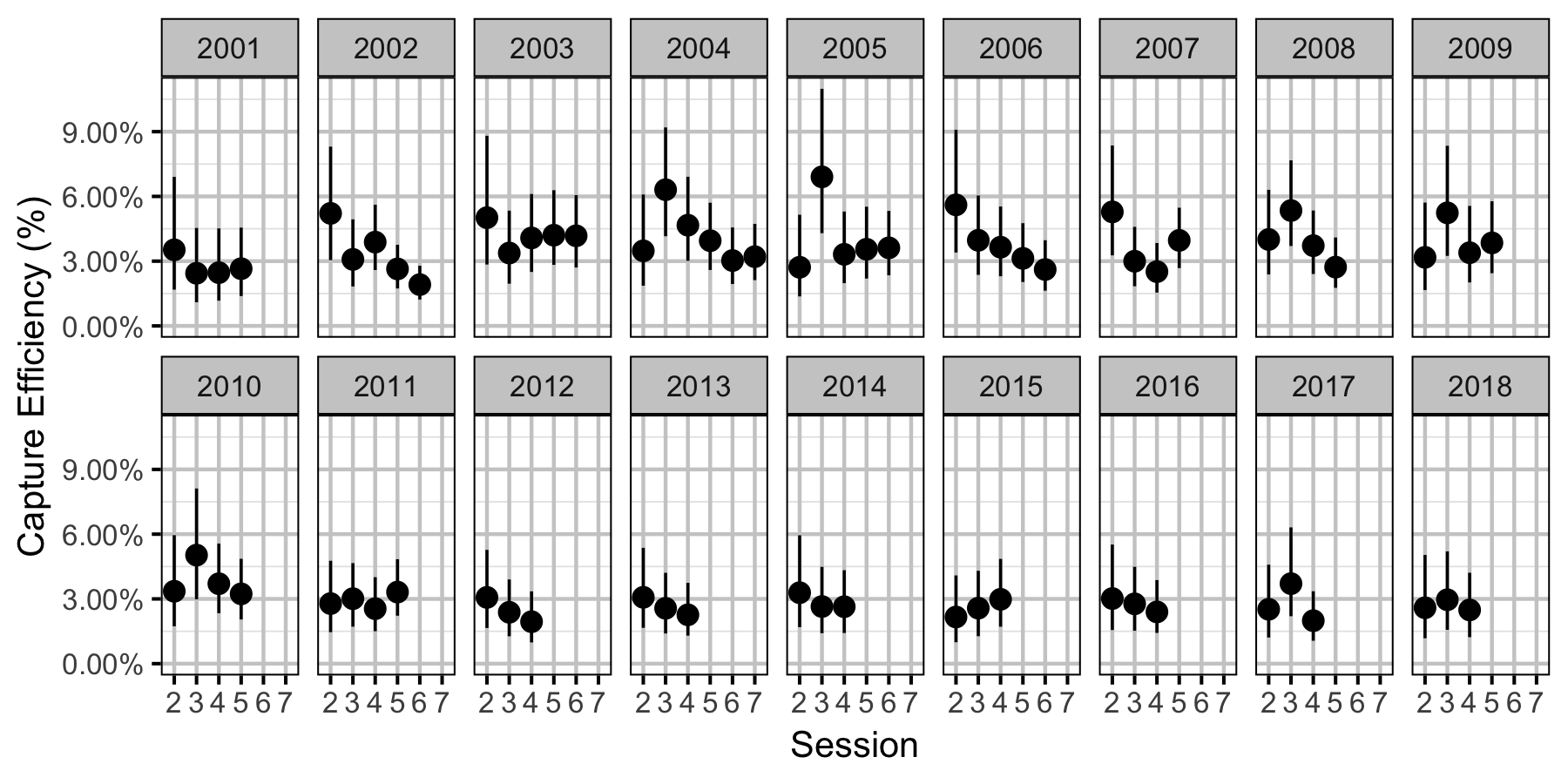
Adult
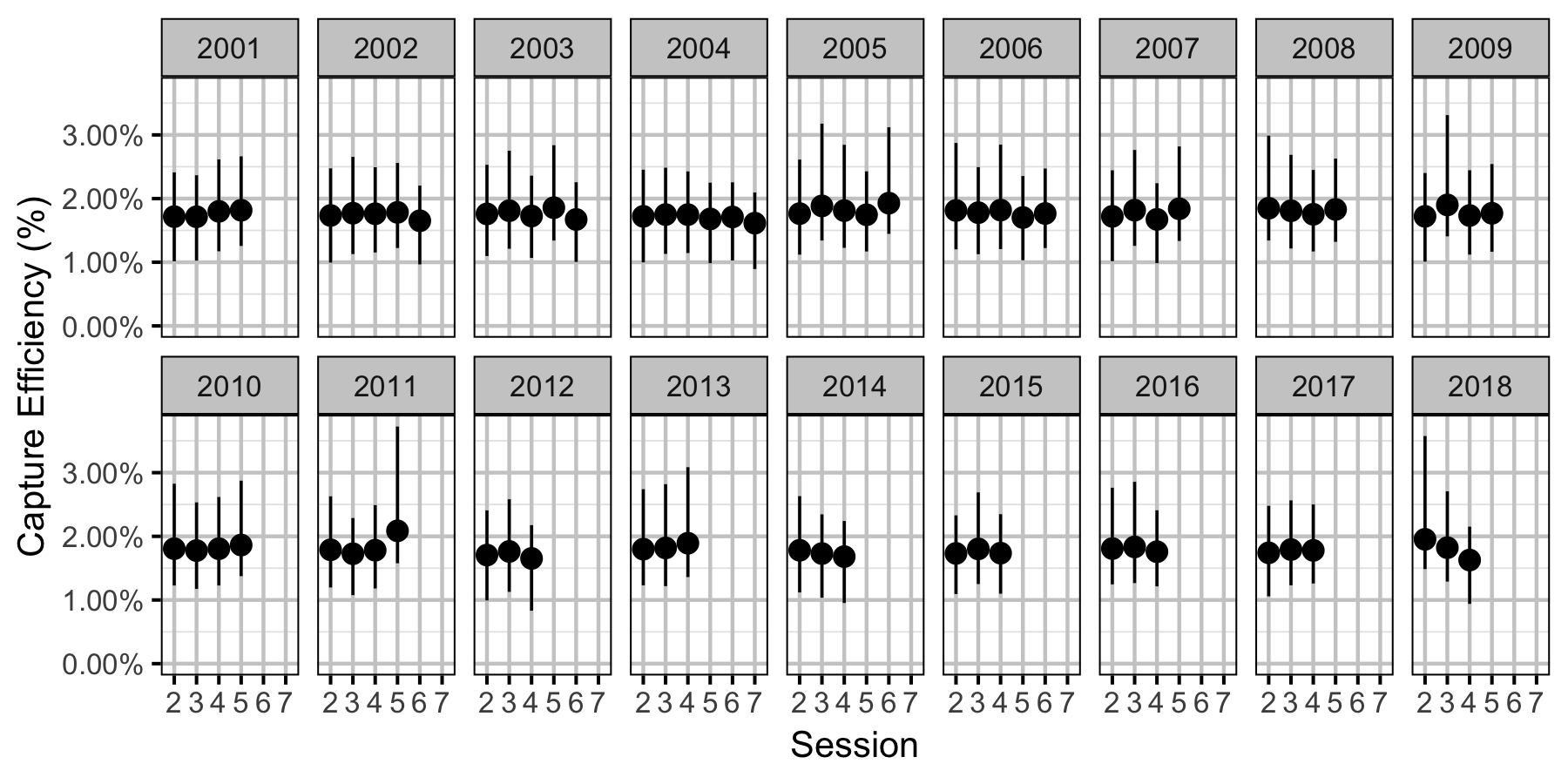
Walleye
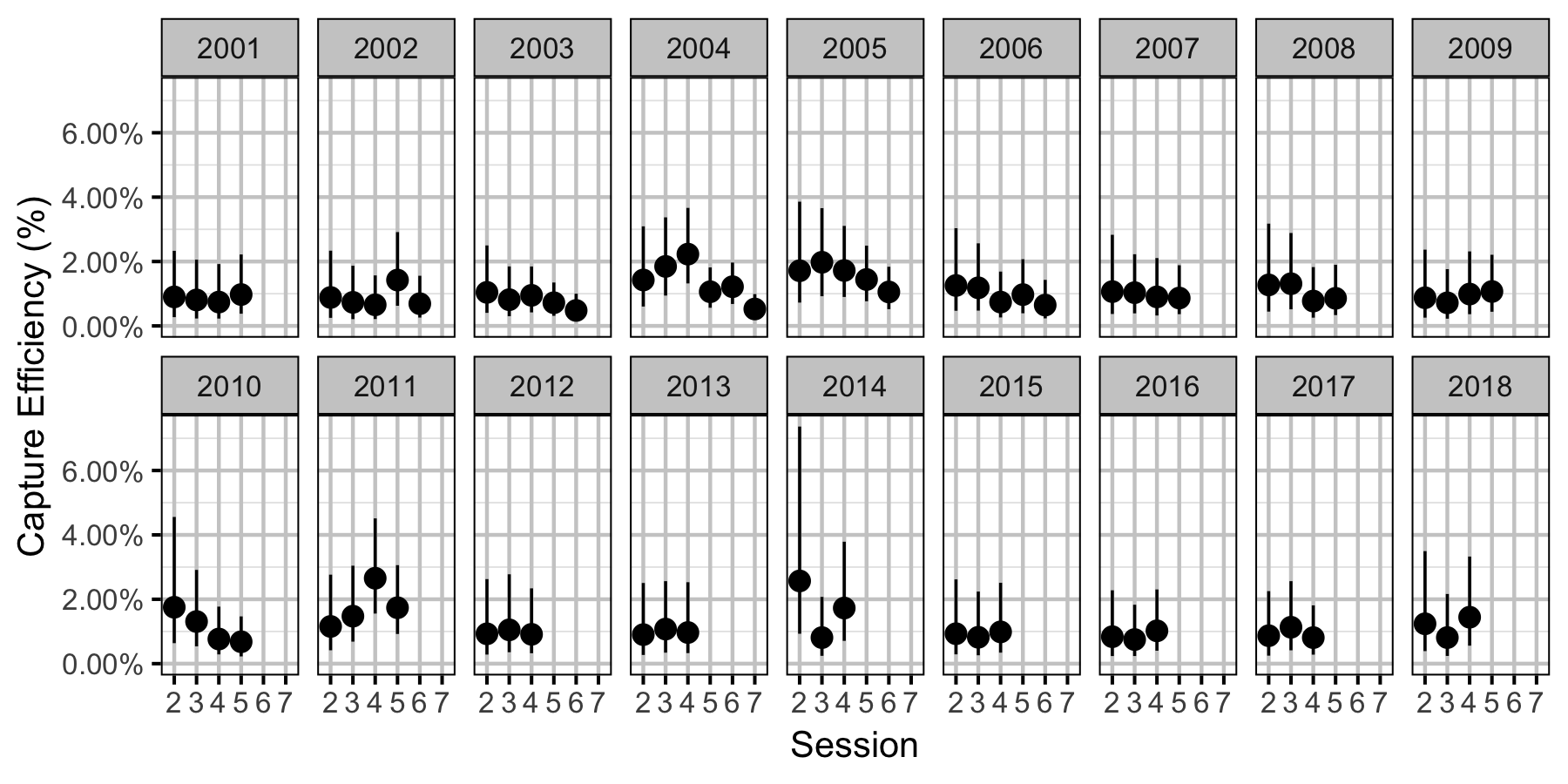
Abundance
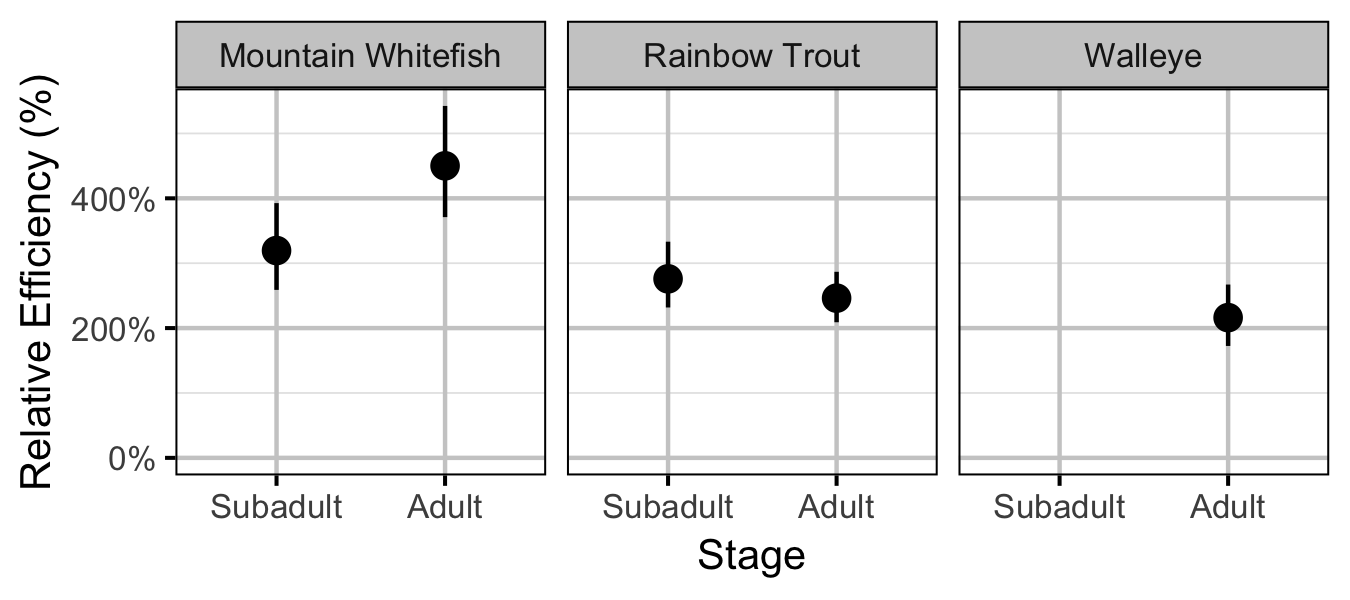
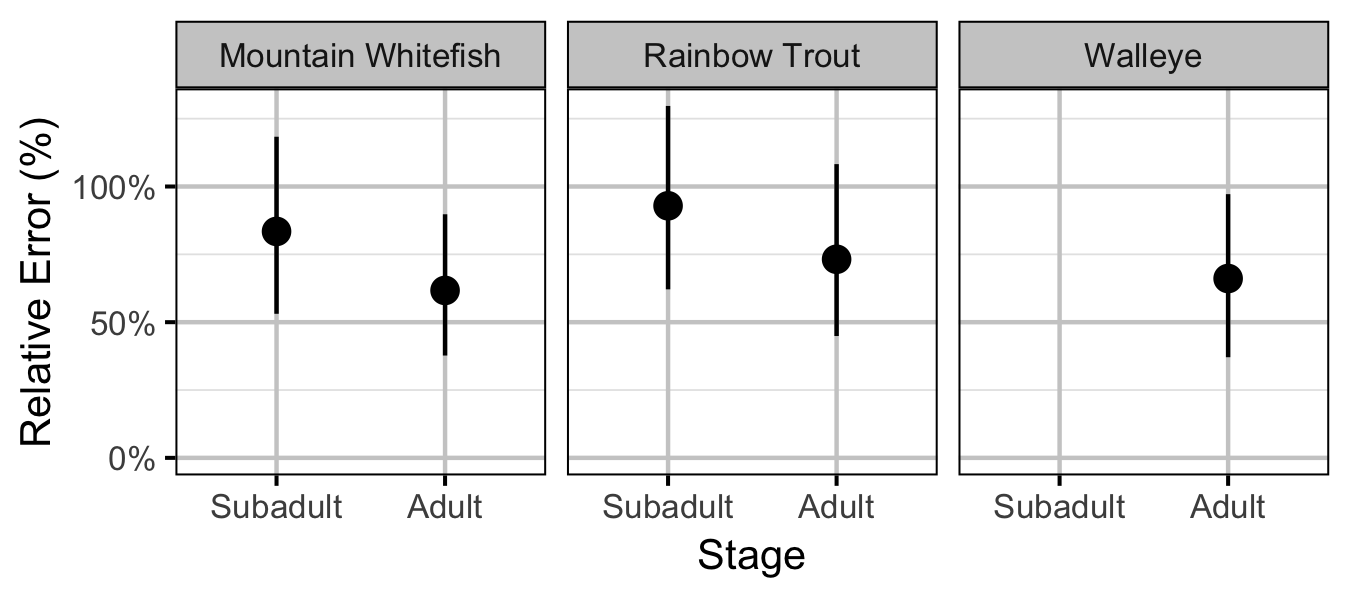
Mountain Whitefish
Subadult
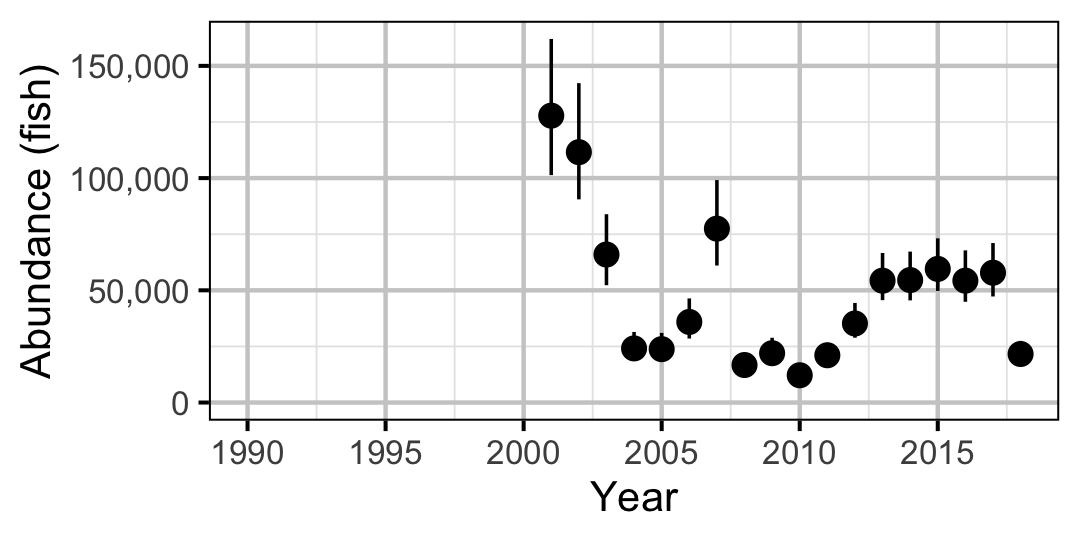
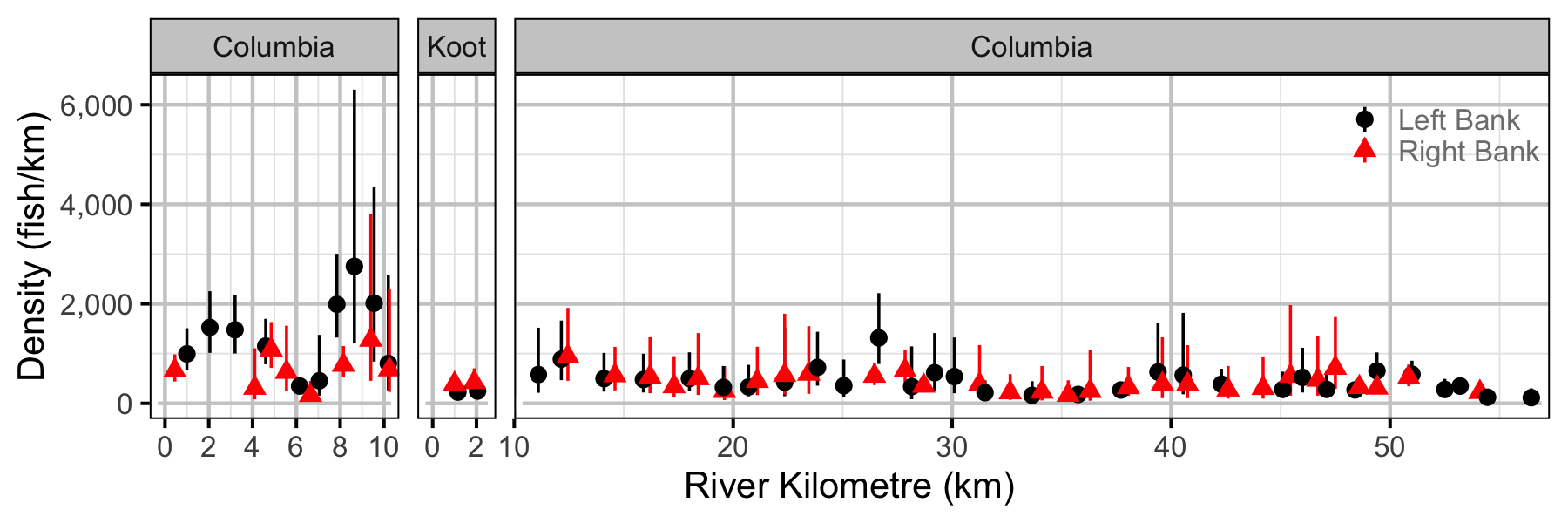
Adult
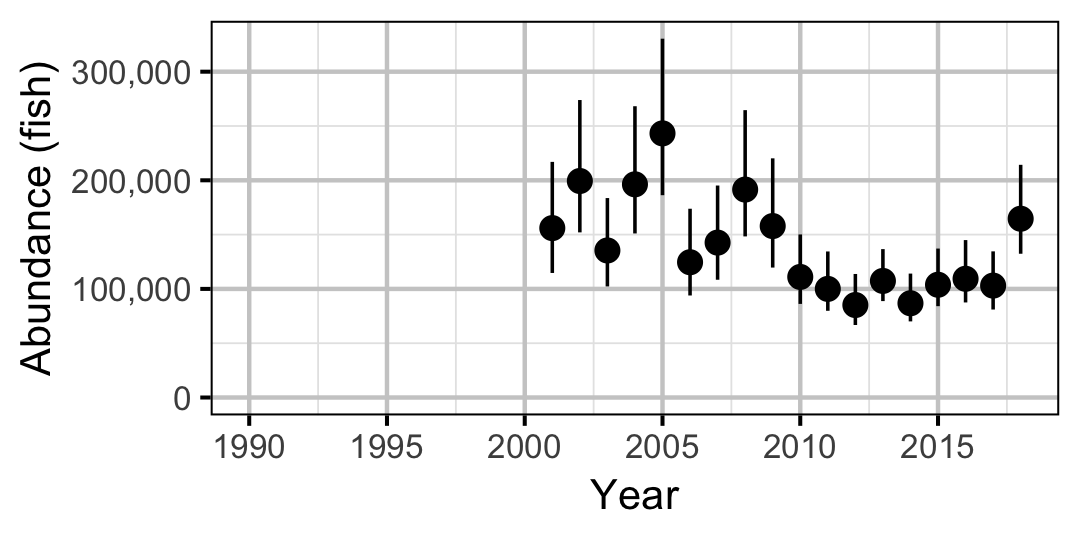
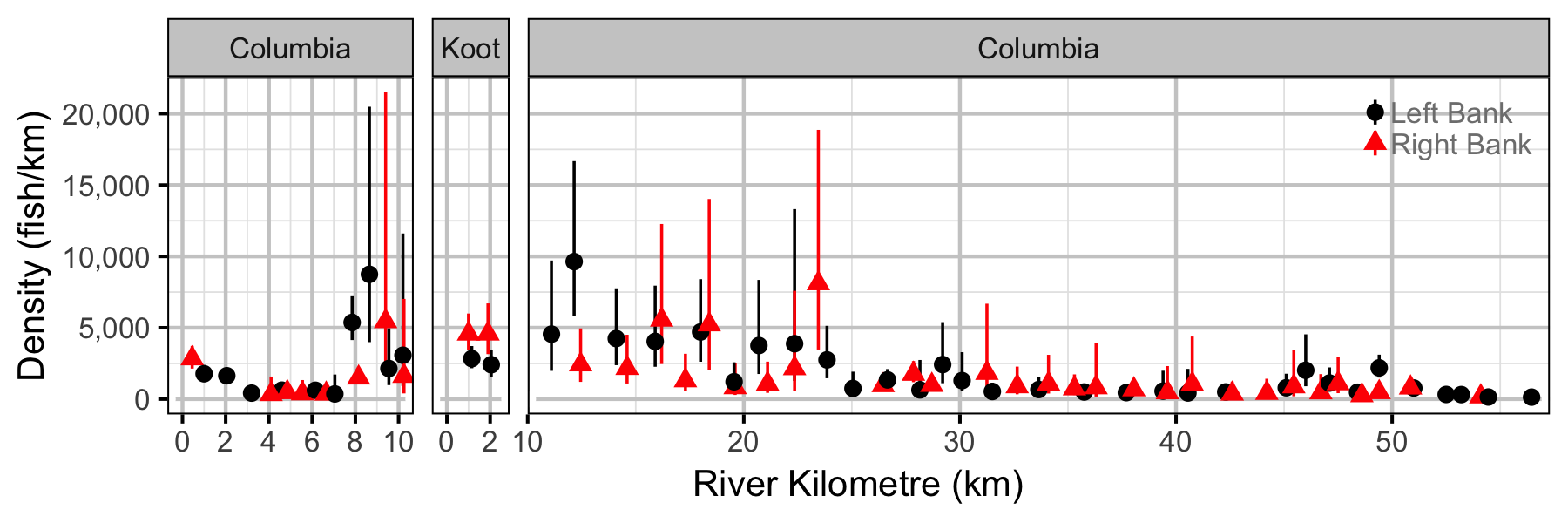
Rainbow Trout
Subadult
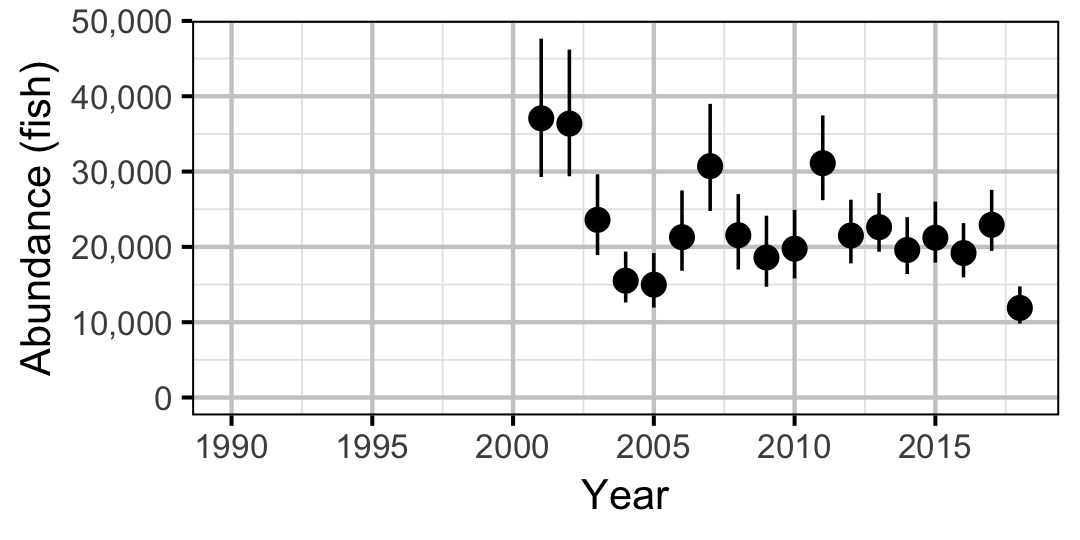
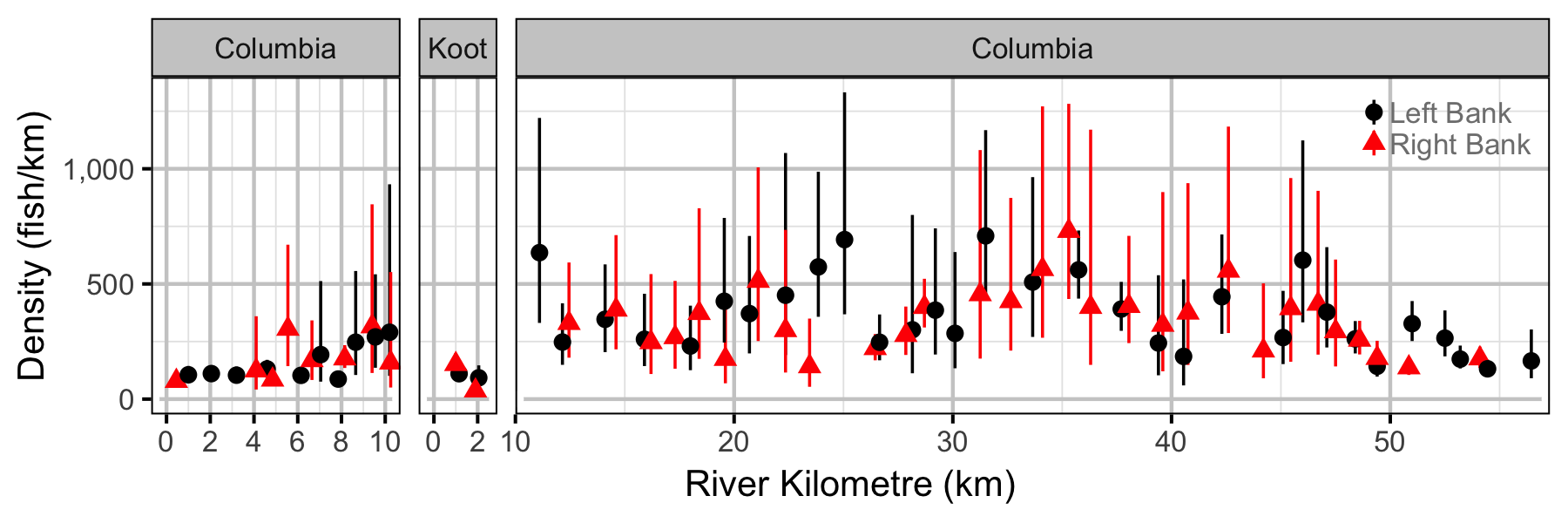
Adult
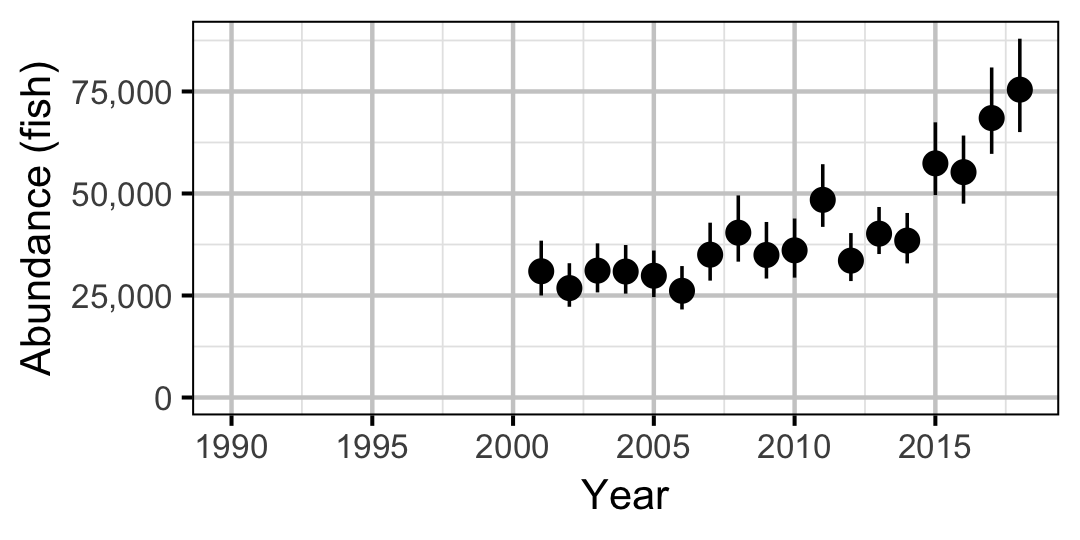
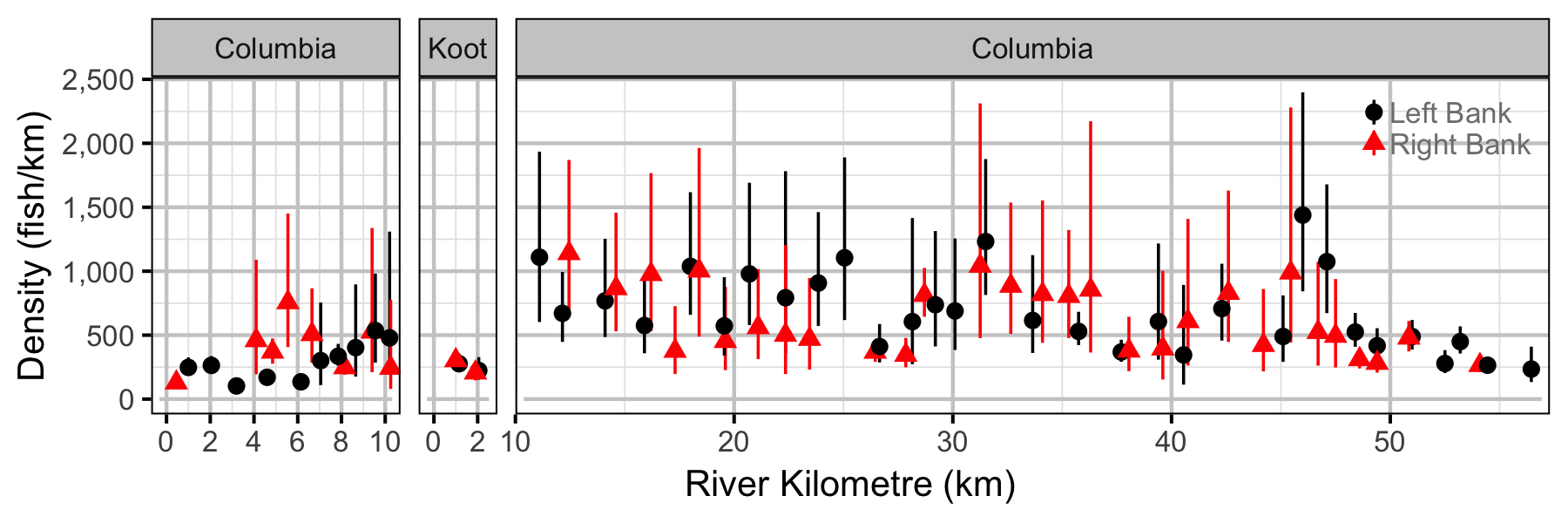
Walleye
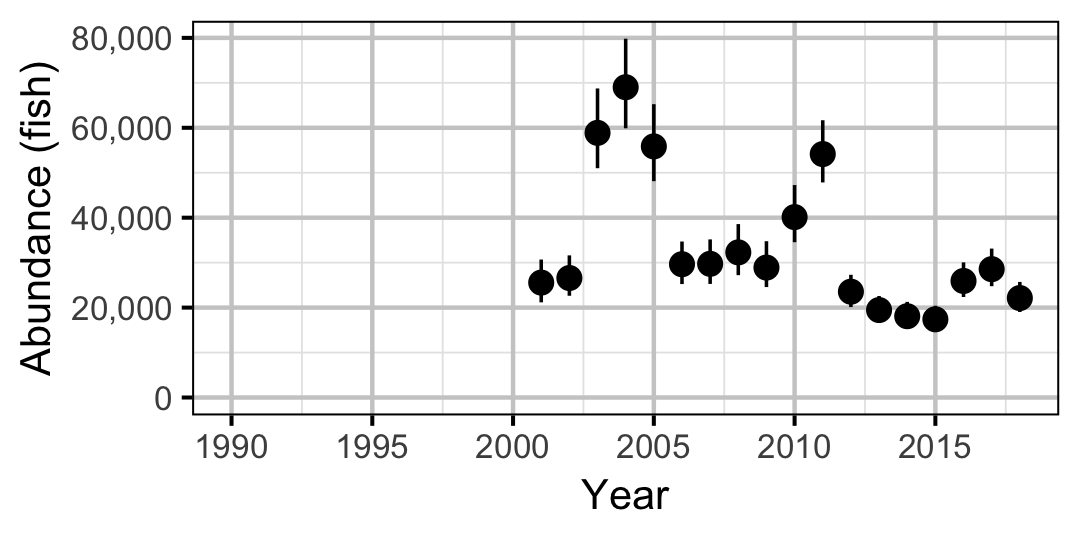
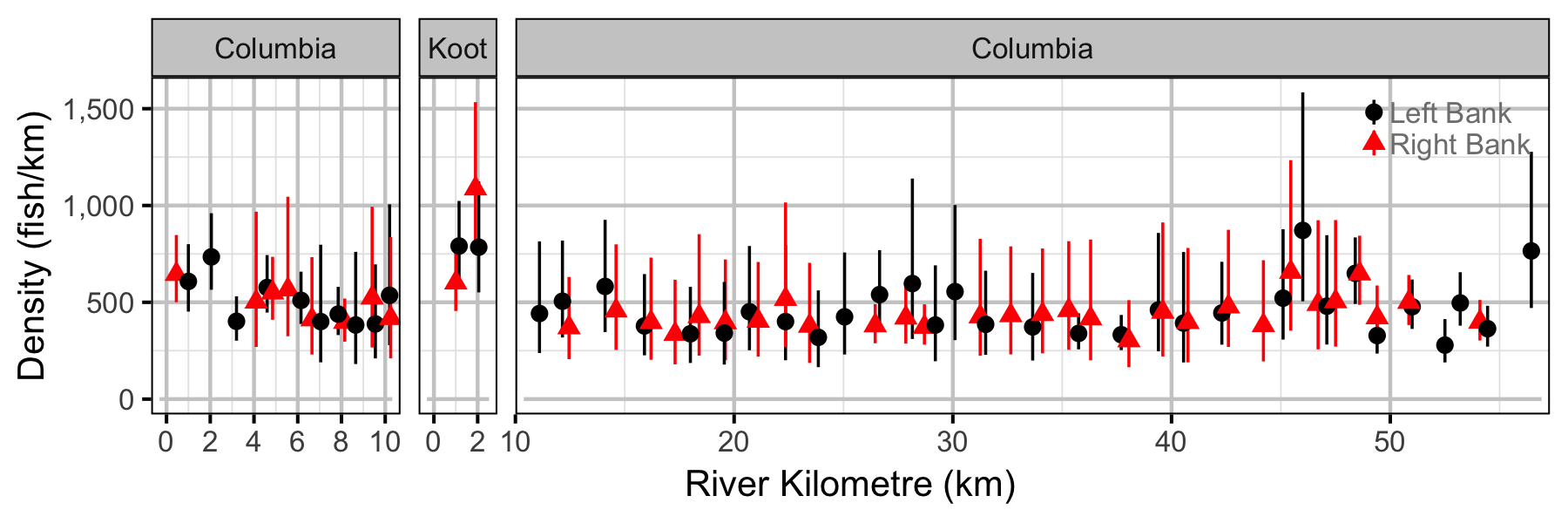
Stock-Recruitment
Mountain Whitefish
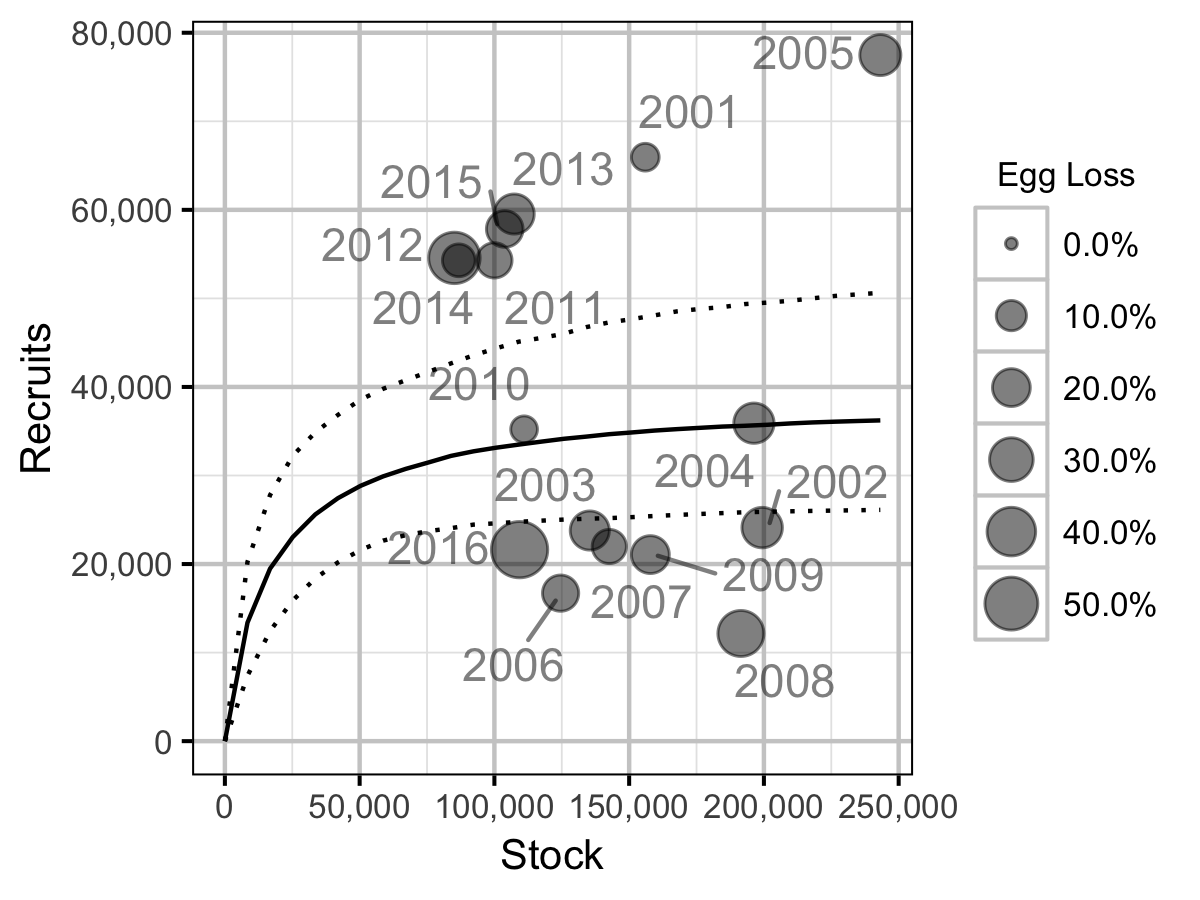
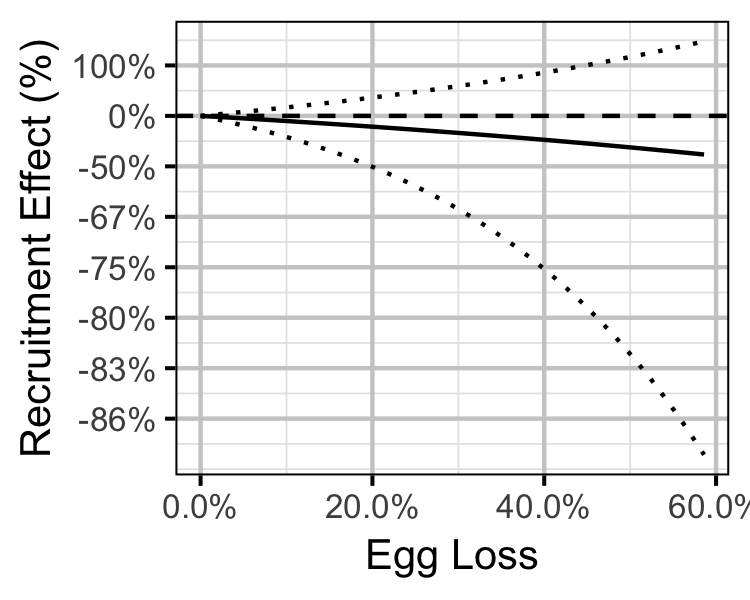
Rainbow Trout
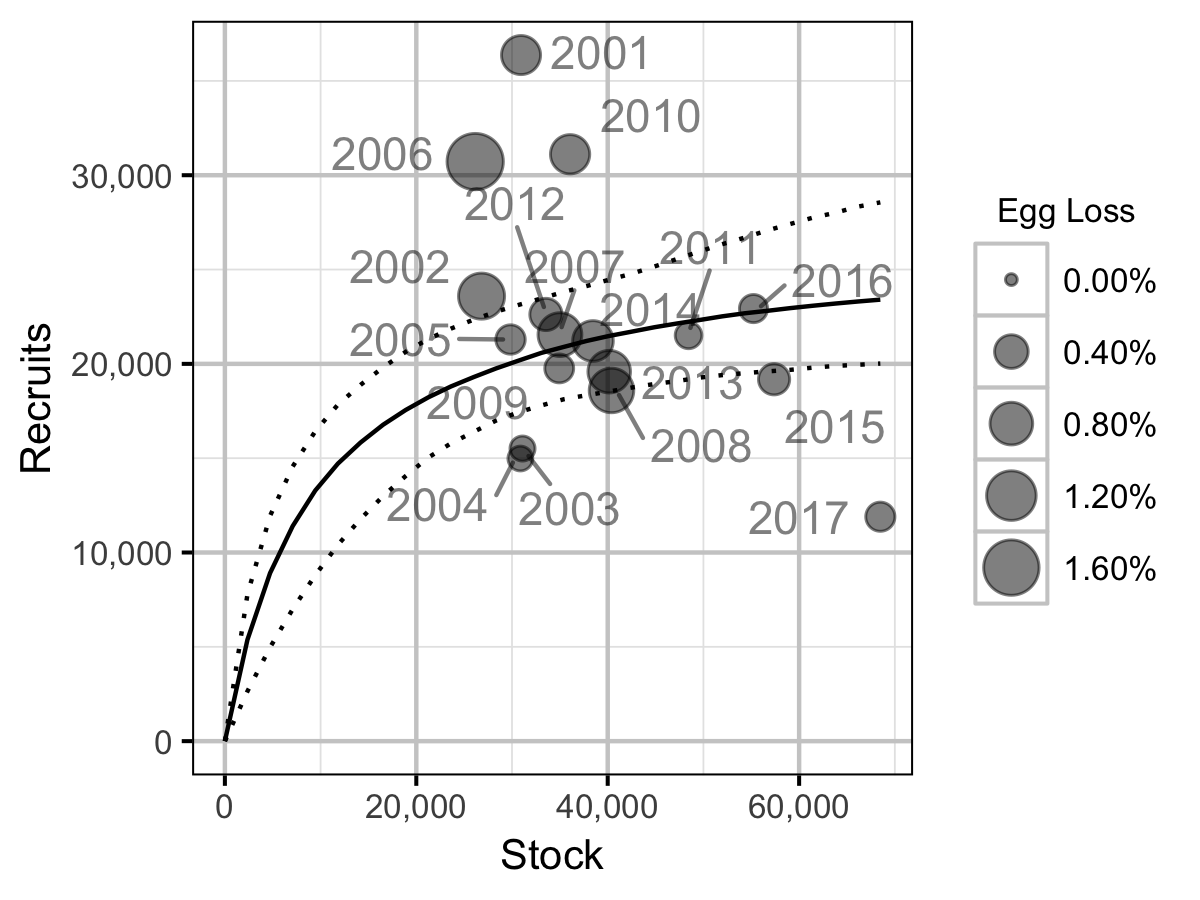
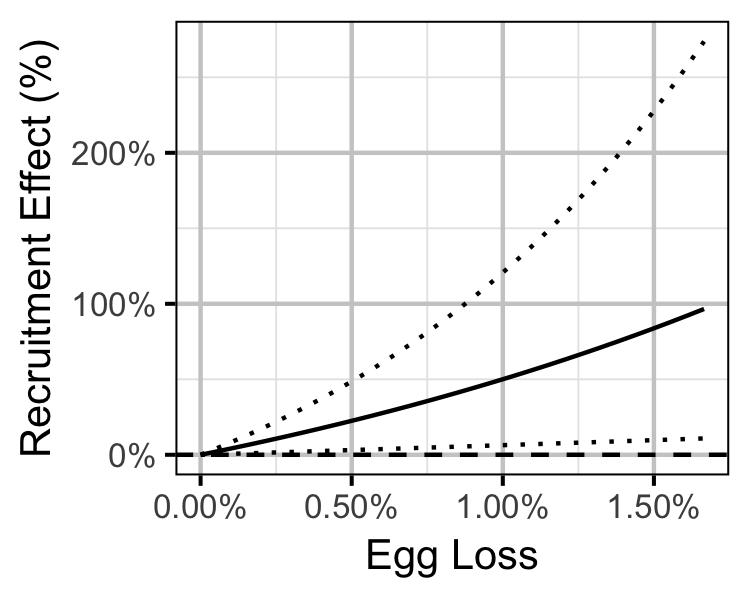
Age-Ratios
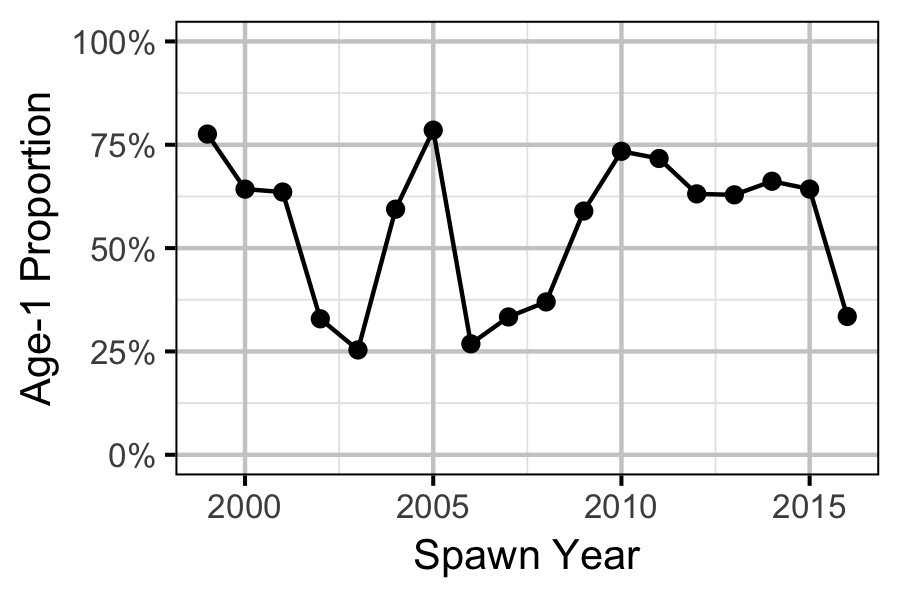
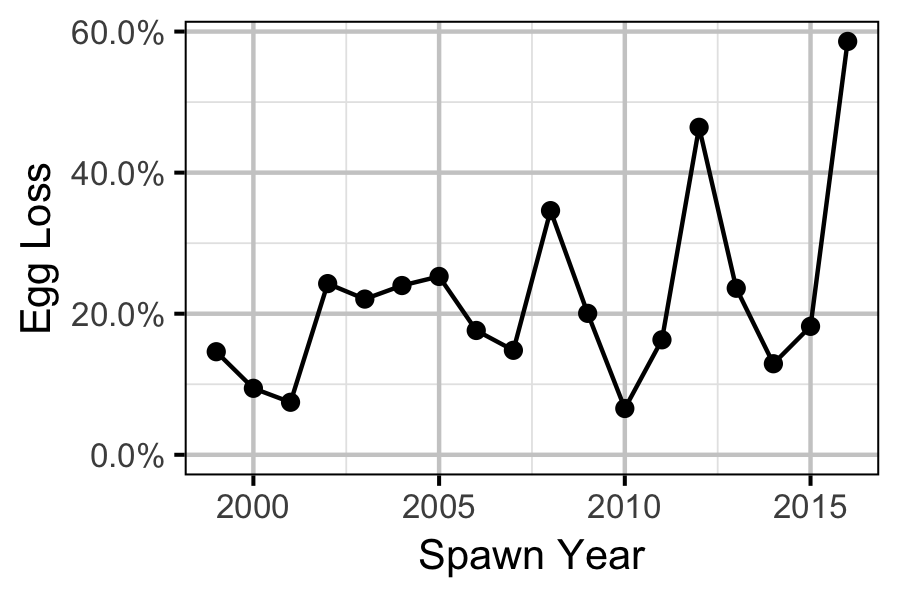
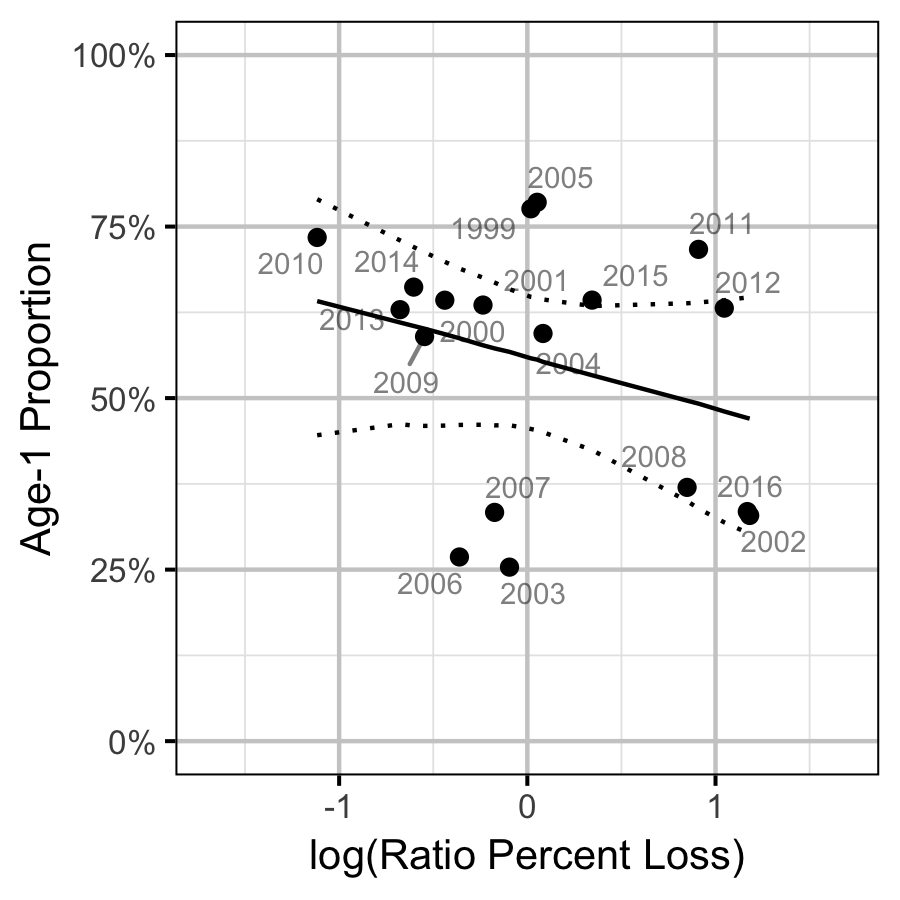
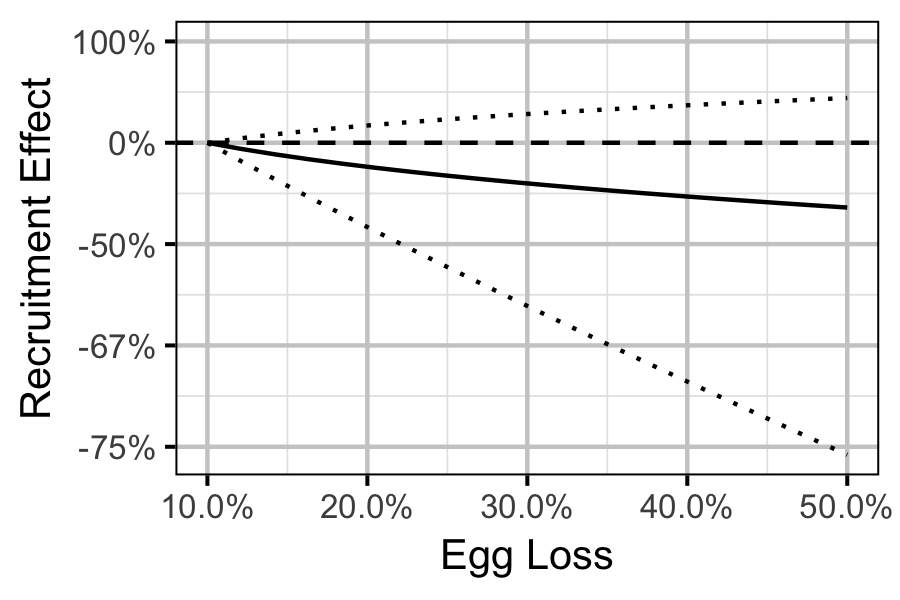
Acknowledgements
The organisations and individuals whose contributions have made this analysis report possible include:
- BC Hydro
- Okanagan Nation Alliance
- Amy Duncan
- Bronwen Lewis
- Golder Associates
- Demitria Burgoon
- Dustin Ford
- David Roscoe
- Sima Usvyatsov
- Dana Schmidt
- Larry Hildebrand
References
Bradford, Michael J, Josh Korman, and Paul S Higgins. 2005. “Using Confidence Intervals to Estimate the Response of Salmon Populations (Oncorhynchus Spp.) to Experimental Habitat Alterations.” Canadian Journal of Fisheries and Aquatic Sciences 62 (12): 2716–26. https://doi.org/10.1139/f05-179.
Brooks, Steve, Andrew Gelman, Galin L. Jones, and Xiao-Li Meng, eds. 2011. Handbook for Markov Chain Monte Carlo. Boca Raton: Taylor & Francis.
Carpenter, Bob, Andrew Gelman, Matthew D. Hoffman, Daniel Lee, Ben Goodrich, Michael Betancourt, Marcus Brubaker, Jiqiang Guo, Peter Li, and Allen Riddell. 2017. “Stan : A Probabilistic Programming Language.” Journal of Statistical Software 76 (1). https://doi.org/10.18637/jss.v076.i01.
Fabens, A J. 1965. “Properties and Fitting of the von Bertalanffy Growth Curve.” Growth 29 (3): 265–89.
Golder Associates Ltd. 2013. “Lower Columbia River Whitefish Spawning Ground Topography Survey: Year 3 Summary Report.” A Golder Associates Ltd. Report 10-1492-0142F. Castlegar, BC: BC Hydro.
He, Ji X., James R. Bence, James E. Johnson, David F. Clapp, and Mark P. Ebener. 2008. “Modeling Variation in Mass-Length Relations and Condition Indices of Lake Trout and Chinook Salmon in Lake Huron: A Hierarchical Bayesian Approach.” Transactions of the American Fisheries Society 137 (3): 801–17. https://doi.org/10.1577/T07-012.1.
Irvine, R. L., J. T. A. Baxter, and J. L. Thorley. 2015. “Lower Columbia River Rainbow Trout Spawning Assessment: Year 7 (2014 Study Period).” A Mountain Water Research and Poisson Consulting Ltd. Report. Castlegar, B.C.: BC Hydro. http://www.bchydro.com/content/dam/BCHydro/customer-portal/documents/corporate/environment-sustainability/water-use-planning/southern-interior/clbmon-46-yr7-2015-02-04.pdf.
Kery, Marc. 2010. Introduction to WinBUGS for Ecologists: A Bayesian Approach to Regression, ANOVA, Mixed Models and Related Analyses. Amsterdam; Boston: Elsevier. http://public.eblib.com/EBLPublic/PublicView.do?ptiID=629953.
Kery, Marc, and Michael Schaub. 2011. Bayesian Population Analysis Using WinBUGS : A Hierarchical Perspective. Boston: Academic Press. http://www.vogelwarte.ch/bpa.html.
Lin, J. 1991. “Divergence Measures Based on the Shannon Entropy.” IEEE Transactions on Information Theory 37 (1): 145–51. https://doi.org/10.1109/18.61115.
Macdonald, P. D. M., and T. J. Pitcher. 1979. “Age-Groups from Size-Frequency Data: A Versatile and Efficient Method of Analyzing Distribution Mixtures.” Journal of the Fisheries Research Board of Canada 36 (8): 987–1001. https://doi.org/10.1139/f79-137.
Macdonald, Peter. 2012. “Mixdist: Finite Mixture Distribution Models.” https://CRAN.R-project.org/package=mixdist.
McElreath, Richard. 2016. Statistical Rethinking: A Bayesian Course with Examples in R and Stan. Chapman & Hall/CRC Texts in Statistical Science Series 122. Boca Raton: CRC Press/Taylor & Francis Group.
Plummer, Martyn. 2015. “JAGS Version 4.0.1 User Manual.” http://sourceforge.net/projects/mcmc-jags/files/Manuals/4.x/.
R Core Team. 2018. R: A Language and Environment for Statistical Computing. Vienna, Austria: R Foundation for Statistical Computing. https://www.R-project.org/.
Subbey, S., J. A. Devine, U. Schaarschmidt, and R. D. M. Nash. 2014. “Modelling and Forecasting Stock-Recruitment: Current and Future Perspectives.” ICES Journal of Marine Science 71 (8): 2307–22. https://doi.org/10.1093/icesjms/fsu148.
Tornqvist, Leo, Pentti Vartia, and Yrjo O. Vartia. 1985. “How Should Relative Changes Be Measured?” The American Statistician 39 (1): 43. https://doi.org/10.2307/2683905.
von Bertalanffy, L. 1938. “A Quantitative Theory of Organic Growth (Inquiries on Growth Laws Ii).” Human Biology 10: 181–213.
Walters, Carl J., and Steven J. D. Martell. 2004. Fisheries Ecology and Management. Princeton, N.J: Princeton University Press.